Docker是开源的应用容器引擎。若想简单了解一下,可以参考百度百科词条Docker。好像只支持64位系统。
Docker官网:https://www.docker.com/
Docker — 从入门到实践:https://yeasy.gitbooks.io/docker_practice/content/
Pdf版下载:http://download.csdn.net/detail/zhangrelay/9743400
caffe官网:http://caffe.berkeleyvision.org/installation.html
caffe_docker:https://github.com/BVLC/caffe/tree/master/docker
然后参考这篇博客就可以了:http://blog.csdn.net/sysushui/article/details/54585788
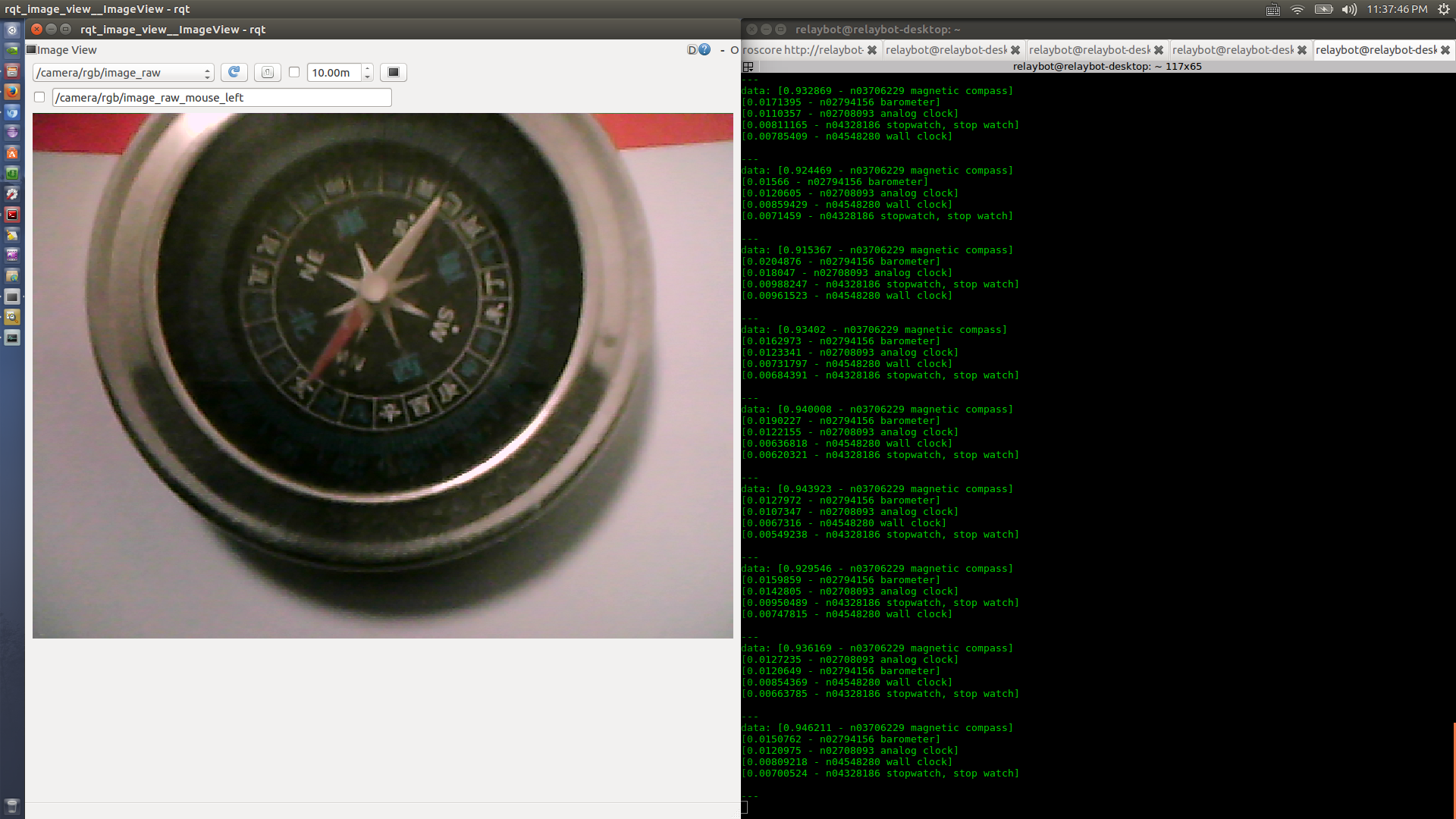
看右图数据,准确识别出是磁罗盘(>0.8)。
如: docker search caffe
$ docker search caffe
NAME DESCRIPTION STARS OFFICIAL AUTOMATED
kaixhin/caffe Ubuntu Core 14.04 + Caffe. 33 [OK]
kaixhin/cuda-caffe Ubuntu Core 14.04 + CUDA + Caffe. 30 [OK]
neowaylabs/caffe-cpu Caffe CPU based on: https://hub.docker.co... 4 [OK]
kaixhin/caffe-deps `kaixhin/caffe` dependencies. 1 [OK]
mbartoli/caffe Caffe, CPU-only 1 [OK]
drunkar/cuda-caffe-anaconda-chainer cuda-caffe-anaconda-chainer 1 [OK]
kaixhin/cuda-caffe-deps `kaixhin/cuda-caffe` dependencies. 0 [OK]
mtngld/caffe-gpu Ubuntu + caffe (gpu ready) 0 [OK]
nitnelave/caffe Master branch of BVLC/caffe, on CentOS7 wi... 0 [OK]
bvlc/caffe Official Caffe images 0 [OK]
ruimashita/caffe-gpu ubuntu 14.04 cuda 7 (NVIDIA driver version... 0 [OK]
ruimashita/caffe-cpu-with-models ubuntu 14.04 caffe bvlc_reference_caffene... 0 [OK]
elezar/caffe Caffe Docker Images 0 [OK]
ruimashita/caffe-gpu-with-models ubuntu 14.04 cuda 7.0 caffe bvlc_referenc... 0 [OK]
floydhub/caffe Caffe docker image 0 [OK]
namikister/caffe Caffe with CUDA 8.0 0 [OK]
tingtinglu/caffe caffe 0 [OK]
djpetti/caffe A simple container with Caffe, CUDA, and C... 0 [OK]
flyingmouse/caffe Caffe is a deep learning framework made wi... 0 [OK]
ruimashita/caffe-cpu ubuntu 14.04 caffe 0 [OK]
suyongsun/caffe-gpu Caffe image with gpu mode. 0 [OK]
haoyangz/caffe-cnn caffe-cnn 0 [OK]
2breakfast/caffe-sshd installed sshd server on nvidia/caffe 0 [OK]
chakkritte/docker-caffe Docker caffe 0 [OK]
ederrm/caffe Caffe http://caffe.berkeleyvision.org setup! 0 [OK]
relaybot@relaybot-desktop:~$
选择安装即可,caffe安装CPU版本还是比较容易的。
安装完毕测试,这是在ros kinetic版本测试,和ros indigo一样。
具体请参考:
http://blog.csdn.net/zhangrelay/article/details/54669922
$ roscore
... logging to /home/relaybot/.ros/log/f214a97a-e0b1-11e6-833d-70f1a1ca7552/roslaunch-relaybot-desktop-32381.log
Checking log directory for disk usage. This may take awhile.
Press Ctrl-C to interrupt
Done checking log file disk usage. Usage is <1GB.
started roslaunch server http://relaybot-desktop:44408/
ros_comm version 1.12.6
SUMMARY
========
PARAMETERS
* /rosdistro: kinetic
* /rosversion: 1.12.6
NODES
auto-starting new master
process[master]: started with pid [32411]
ROS_MASTER_URI=http://relaybot-desktop:11311/
setting /run_id to f214a97a-e0b1-11e6-833d-70f1a1ca7552
process[rosout-1]: started with pid [32424]
started core service [/rosout]
$ rosrun uvc_camera uvc_camera_node
[ INFO] [1485096579.984543774]: using default calibration URL
[ INFO] [1485096579.984671839]: camera calibration URL: file:///home/relaybot/.ros/camera_info/camera.yaml
[ INFO] [1485096579.984939036]: Unable to open camera calibration file [/home/relaybot/.ros/camera_info/camera.yaml]
[ WARN] [1485096579.984987494]: Camera calibration file /home/relaybot/.ros/camera_info/camera.yaml not found.
opening /dev/video0
pixfmt 0 = 'YUYV' desc = 'YUYV 4:2:2'
discrete: 640x480: 1/30 1/15
discrete: 352x288: 1/30 1/15
discrete: 320x240: 1/30 1/15
discrete: 176x144: 1/30 1/15
discrete: 160x120: 1/30 1/15
discrete: 1280x800: 2/15
discrete: 1280x1024: 2/15
int (Brightness, 0, id = 980900): -64 to 64 (1)
int (Contrast, 0, id = 980901): 0 to 64 (1)
int (Saturation, 0, id = 980902): 0 to 128 (1)
int (Hue, 0, id = 980903): -40 to 40 (1)
bool (White Balance Temperature, Auto, 0, id = 98090c): 0 to 1 (1)
int (Gamma, 0, id = 980910): 72 to 500 (1)
menu (Power Line Frequency, 0, id = 980918): 0 to 2 (1)
0: Disabled
1: 50 Hz
2: 60 Hz
int (Sharpness, 0, id = 98091b): 0 to 6 (1)
int (Backlight Compensation, 0, id = 98091c): 0 to 2 (1)
select timeout in grab
^Crelaybot@relaybot-desktop:~$ rosrun uvc_camera uvc_camera_node topic:=/camera/b/image_raw
[ INFO] [1485096761.665718381]: using default calibration URL
[ INFO] [1485096761.665859706]: camera calibration URL: file:///home/relaybot/.ros/camera_info/camera.yaml
[ INFO] [1485096761.665944994]: Unable to open camera calibration file [/home/relaybot/.ros/camera_info/camera.yaml]
[ WARN] [1485096761.665980436]: Camera calibration file /home/relaybot/.ros/camera_info/camera.yaml not found.
opening /dev/video0
pixfmt 0 = 'YUYV' desc = 'YUYV 4:2:2'
discrete: 640x480: 1/30 1/15
discrete: 352x288: 1/30 1/15
discrete: 320x240: 1/30 1/15
discrete: 176x144: 1/30 1/15
discrete: 160x120: 1/30 1/15
discrete: 1280x800: 2/15
discrete: 1280x1024: 2/15
int (Brightness, 0, id = 980900): -64 to 64 (1)
int (Contrast, 0, id = 980901): 0 to 64 (1)
int (Saturation, 0, id = 980902): 0 to 128 (1)
int (Hue, 0, id = 980903): -40 to 40 (1)
bool (White Balance Temperature, Auto, 0, id = 98090c): 0 to 1 (1)
int (Gamma, 0, id = 980910): 72 to 500 (1)
menu (Power Line Frequency, 0, id = 980918): 0 to 2 (1)
0: Disabled
1: 50 Hz
2: 60 Hz
int (Sharpness, 0, id = 98091b): 0 to 6 (1)
int (Backlight Compensation, 0, id = 98091c): 0 to 2 (1)
select timeout in grab
rosrun topic_tools transform /image_raw /camera/rgb/image_raw sensor_msgs/Image 'm'
$ rosrun ros_caffe ros_caffe_test
WARNING: Logging before InitGoogleLogging() is written to STDERR
I0122 23:02:21.915738 2968 upgrade_proto.cpp:67] Attempting to upgrade input file specified using deprecated input fields: /home/relaybot/Rob_Soft/caffe/src/ros_caffe/data/deploy.prototxt
I0122 23:02:21.915875 2968 upgrade_proto.cpp:70] Successfully upgraded file specified using deprecated input fields.
W0122 23:02:21.915894 2968 upgrade_proto.cpp:72] Note that future Caffe releases will only support input layers and not input fields.
I0122 23:02:21.916246 2968 net.cpp:53] Initializing net from parameters:
name: "CaffeNet"
state {
phase: TEST
level: 0
}
layer {
name: "input"
type: "Input"
top: "data"
input_param {
shape {
dim: 10
dim: 3
dim: 227
dim: 227
}
}
}
layer {
name: "conv1"
type: "Convolution"
bottom: "data"
top: "conv1"
convolution_param {
num_output: 96
kernel_size: 11
stride: 4
}
}
layer {
name: "relu1"
type: "ReLU"
bottom: "conv1"
top: "conv1"
}
layer {
name: "pool1"
type: "Pooling"
bottom: "conv1"
top: "pool1"
pooling_param {
pool: MAX
kernel_size: 3
stride: 2
}
}
layer {
name: "norm1"
type: "LRN"
bottom: "pool1"
top: "norm1"
lrn_param {
local_size: 5
alpha: 0.0001
beta: 0.75
}
}
layer {
name: "conv2"
type: "Convolution"
bottom: "norm1"
top: "conv2"
convolution_param {
num_output: 256
pad: 2
kernel_size: 5
group: 2
}
}
layer {
name: "relu2"
type: "ReLU"
bottom: "conv2"
top: "conv2"
}
layer {
name: "pool2"
type: "Pooling"
bottom: "conv2"
top: "pool2"
pooling_param {
pool: MAX
kernel_size: 3
stride: 2
}
}
layer {
name: "norm2"
type: "LRN"
bottom: "pool2"
top: "norm2"
lrn_param {
local_size: 5
alpha: 0.0001
beta: 0.75
}
}
layer {
name: "conv3"
type: "Convolution"
bottom: "norm2"
top: "conv3"
convolution_param {
num_output: 384
pad: 1
kernel_size: 3
}
}
layer {
name: "relu3"
type: "ReLU"
bottom: "conv3"
top: "conv3"
}
layer {
name: "conv4"
type: "Convolution"
bottom: "conv3"
top: "conv4"
convolution_param {
num_output: 384
pad: 1
kernel_size: 3
group: 2
}
}
layer {
name: "relu4"
type: "ReLU"
bottom: "conv4"
top: "conv4"
}
layer {
name: "conv5"
type: "Convolution"
bottom: "conv4"
top: "conv5"
convolution_param {
num_output: 256
pad: 1
kernel_size: 3
group: 2
}
}
layer {
name: "relu5"
type: "ReLU"
bottom: "conv5"
top: "conv5"
}
layer {
name: "pool5"
type: "Pooling"
bottom: "conv5"
top: "pool5"
pooling_param {
pool: MAX
kernel_size: 3
stride: 2
}
}
layer {
name: "fc6"
type: "InnerProduct"
bottom: "pool5"
top: "fc6"
inner_product_param {
num_output: 4096
}
}
layer {
name: "relu6"
type: "ReLU"
bottom: "fc6"
top: "fc6"
}
layer {
name: "drop6"
type: "Dropout"
bottom: "fc6"
top: "fc6"
dropout_param {
dropout_ratio: 0.5
}
}
layer {
name: "fc7"
type: "InnerProduct"
bottom: "fc6"
top: "fc7"
inner_product_param {
num_output: 4096
}
}
layer {
name: "relu7"
type: "ReLU"
bottom: "fc7"
top: "fc7"
}
layer {
name: "drop7"
type: "Dropout"
bottom: "fc7"
top: "fc7"
dropout_param {
dropout_ratio: 0.5
}
}
layer {
name: "fc8"
type: "InnerProduct"
bottom: "fc7"
top: "fc8"
inner_product_param {
num_output: 1000
}
}
layer {
name: "prob"
type: "Softmax"
bottom: "fc8"
top: "prob"
}
I0122 23:02:21.916574 2968 layer_factory.hpp:77] Creating layer input
I0122 23:02:21.916613 2968 net.cpp:86] Creating Layer input
I0122 23:02:21.916638 2968 net.cpp:382] input -> data
I0122 23:02:21.931437 2968 net.cpp:124] Setting up input
I0122 23:02:21.939075 2968 net.cpp:131] Top shape: 10 3 227 227 (1545870)
I0122 23:02:21.939122 2968 net.cpp:139] Memory required for data: 6183480
I0122 23:02:21.939157 2968 layer_factory.hpp:77] Creating layer conv1
I0122 23:02:21.939210 2968 net.cpp:86] Creating Layer conv1
I0122 23:02:21.939235 2968 net.cpp:408] conv1 <- data
I0122 23:02:21.939278 2968 net.cpp:382] conv1 -> conv1
I0122 23:02:21.939563 2968 net.cpp:124] Setting up conv1
I0122 23:02:21.939604 2968 net.cpp:131] Top shape: 10 96 55 55 (2904000)
I0122 23:02:21.939618 2968 net.cpp:139] Memory required for data: 17799480
I0122 23:02:21.939685 2968 layer_factory.hpp:77] Creating layer relu1
I0122 23:02:21.939714 2968 net.cpp:86] Creating Layer relu1
I0122 23:02:21.939730 2968 net.cpp:408] relu1 <- conv1
I0122 23:02:21.939752 2968 net.cpp:369] relu1 -> conv1 (in-place)
I0122 23:02:21.939781 2968 net.cpp:124] Setting up relu1
I0122 23:02:21.939802 2968 net.cpp:131] Top shape: 10 96 55 55 (2904000)
I0122 23:02:21.939817 2968 net.cpp:139] Memory required for data: 29415480
I0122 23:02:21.939832 2968 layer_factory.hpp:77] Creating layer pool1
I0122 23:02:21.939857 2968 net.cpp:86] Creating Layer pool1
I0122 23:02:21.939868 2968 net.cpp:408] pool1 <- conv1
I0122 23:02:21.939887 2968 net.cpp:382] pool1 -> pool1
I0122 23:02:21.939947 2968 net.cpp:124] Setting up pool1
I0122 23:02:21.939967 2968 net.cpp:131] Top shape: 10 96 27 27 (699840)
I0122 23:02:21.939980 2968 net.cpp:139] Memory required for data: 32214840
I0122 23:02:21.939992 2968 layer_factory.hpp:77] Creating layer norm1
I0122 23:02:21.940014 2968 net.cpp:86] Creating Layer norm1
I0122 23:02:21.940027 2968 net.cpp:408] norm1 <- pool1
I0122 23:02:21.940045 2968 net.cpp:382] norm1 -> norm1
I0122 23:02:21.940075 2968 net.cpp:124] Setting up norm1
I0122 23:02:21.940093 2968 net.cpp:131] Top shape: 10 96 27 27 (699840)
I0122 23:02:21.940104 2968 net.cpp:139] Memory required for data: 35014200
I0122 23:02:21.940116 2968 layer_factory.hpp:77] Creating layer conv2
I0122 23:02:21.940137 2968 net.cpp:86] Creating Layer conv2
I0122 23:02:21.940152 2968 net.cpp:408] conv2 <- norm1
I0122 23:02:21.940171 2968 net.cpp:382] conv2 -> conv2
I0122 23:02:21.940996 2968 net.cpp:124] Setting up conv2
I0122 23:02:21.941033 2968 net.cpp:131] Top shape: 10 256 27 27 (1866240)
I0122 23:02:21.941045 2968 net.cpp:139] Memory required for data: 42479160
I0122 23:02:21.941121 2968 layer_factory.hpp:77] Creating layer relu2
I0122 23:02:21.941144 2968 net.cpp:86] Creating Layer relu2
I0122 23:02:21.941157 2968 net.cpp:408] relu2 <- conv2
I0122 23:02:21.941174 2968 net.cpp:369] relu2 -> conv2 (in-place)
I0122 23:02:21.941193 2968 net.cpp:124] Setting up relu2
I0122 23:02:21.941208 2968 net.cpp:131] Top shape: 10 256 27 27 (1866240)
I0122 23:02:21.941220 2968 net.cpp:139] Memory required for data: 49944120
I0122 23:02:21.941232 2968 layer_factory.hpp:77] Creating layer pool2
I0122 23:02:21.941248 2968 net.cpp:86] Creating Layer pool2
I0122 23:02:21.941259 2968 net.cpp:408] pool2 <- conv2
I0122 23:02:21.941275 2968 net.cpp:382] pool2 -> pool2
I0122 23:02:21.941301 2968 net.cpp:124] Setting up pool2
I0122 23:02:21.941316 2968 net.cpp:131] Top shape: 10 256 13 13 (432640)
I0122 23:02:21.941328 2968 net.cpp:139] Memory required for data: 51674680
I0122 23:02:21.941339 2968 layer_factory.hpp:77] Creating layer norm2
I0122 23:02:21.941360 2968 net.cpp:86] Creating Layer norm2
I0122 23:02:21.941372 2968 net.cpp:408] norm2 <- pool2
I0122 23:02:21.941390 2968 net.cpp:382] norm2 -> norm2
I0122 23:02:21.941411 2968 net.cpp:124] Setting up norm2
I0122 23:02:21.941426 2968 net.cpp:131] Top shape: 10 256 13 13 (432640)
I0122 23:02:21.941437 2968 net.cpp:139] Memory required for data: 53405240
I0122 23:02:21.941448 2968 layer_factory.hpp:77] Creating layer conv3
I0122 23:02:21.941468 2968 net.cpp:86] Creating Layer conv3
I0122 23:02:21.941478 2968 net.cpp:408] conv3 <- norm2
I0122 23:02:21.941495 2968 net.cpp:382] conv3 -> conv3
I0122 23:02:21.943603 2968 net.cpp:124] Setting up conv3
I0122 23:02:21.943662 2968 net.cpp:131] Top shape: 10 384 13 13 (648960)
I0122 23:02:21.943675 2968 net.cpp:139] Memory required for data: 56001080
I0122 23:02:21.943711 2968 layer_factory.hpp:77] Creating layer relu3
I0122 23:02:21.943733 2968 net.cpp:86] Creating Layer relu3
I0122 23:02:21.943747 2968 net.cpp:408] relu3 <- conv3
I0122 23:02:21.943765 2968 net.cpp:369] relu3 -> conv3 (in-place)
I0122 23:02:21.943786 2968 net.cpp:124] Setting up relu3
I0122 23:02:21.943801 2968 net.cpp:131] Top shape: 10 384 13 13 (648960)
I0122 23:02:21.943812 2968 net.cpp:139] Memory required for data: 58596920
I0122 23:02:21.943822 2968 layer_factory.hpp:77] Creating layer conv4
I0122 23:02:21.943848 2968 net.cpp:86] Creating Layer conv4
I0122 23:02:21.943861 2968 net.cpp:408] conv4 <- conv3
I0122 23:02:21.943881 2968 net.cpp:382] conv4 -> conv4
I0122 23:02:21.944964 2968 net.cpp:124] Setting up conv4
I0122 23:02:21.945030 2968 net.cpp:131] Top shape: 10 384 13 13 (648960)
I0122 23:02:21.945047 2968 net.cpp:139] Memory required for data: 61192760
I0122 23:02:21.945148 2968 layer_factory.hpp:77] Creating layer relu4
I0122 23:02:21.945188 2968 net.cpp:86] Creating Layer relu4
I0122 23:02:21.945206 2968 net.cpp:408] relu4 <- conv4
I0122 23:02:21.945230 2968 net.cpp:369] relu4 -> conv4 (in-place)
I0122 23:02:21.945258 2968 net.cpp:124] Setting up relu4
I0122 23:02:21.945277 2968 net.cpp:131] Top shape: 10 384 13 13 (648960)
I0122 23:02:21.945291 2968 net.cpp:139] Memory required for data: 63788600
I0122 23:02:21.945303 2968 layer_factory.hpp:77] Creating layer conv5
I0122 23:02:21.945334 2968 net.cpp:86] Creating Layer conv5
I0122 23:02:21.945353 2968 net.cpp:408] conv5 <- conv4
I0122 23:02:21.945376 2968 net.cpp:382] conv5 -> conv5
I0122 23:02:21.946549 2968 net.cpp:124] Setting up conv5
I0122 23:02:21.946606 2968 net.cpp:131] Top shape: 10 256 13 13 (432640)
I0122 23:02:21.946622 2968 net.cpp:139] Memory required for data: 65519160
I0122 23:02:21.946672 2968 layer_factory.hpp:77] Creating layer relu5
I0122 23:02:21.946698 2968 net.cpp:86] Creating Layer relu5
I0122 23:02:21.946717 2968 net.cpp:408] relu5 <- conv5
I0122 23:02:21.946743 2968 net.cpp:369] relu5 -> conv5 (in-place)
I0122 23:02:21.946771 2968 net.cpp:124] Setting up relu5
I0122 23:02:21.946792 2968 net.cpp:131] Top shape: 10 256 13 13 (432640)
I0122 23:02:21.946812 2968 net.cpp:139] Memory required for data: 67249720
I0122 23:02:21.946826 2968 layer_factory.hpp:77] Creating layer pool5
I0122 23:02:21.946848 2968 net.cpp:86] Creating Layer pool5
I0122 23:02:21.946864 2968 net.cpp:408] pool5 <- conv5
I0122 23:02:21.946885 2968 net.cpp:382] pool5 -> pool5
I0122 23:02:21.946935 2968 net.cpp:124] Setting up pool5
I0122 23:02:21.946971 2968 net.cpp:131] Top shape: 10 256 6 6 (92160)
I0122 23:02:21.946986 2968 net.cpp:139] Memory required for data: 67618360
I0122 23:02:21.947003 2968 layer_factory.hpp:77] Creating layer fc6
I0122 23:02:21.947028 2968 net.cpp:86] Creating Layer fc6
I0122 23:02:21.947044 2968 net.cpp:408] fc6 <- pool5
I0122 23:02:21.947065 2968 net.cpp:382] fc6 -> fc6
I0122 23:02:21.989847 2968 net.cpp:124] Setting up fc6
I0122 23:02:21.989913 2968 net.cpp:131] Top shape: 10 4096 (40960)
I0122 23:02:21.989919 2968 net.cpp:139] Memory required for data: 67782200
I0122 23:02:21.989943 2968 layer_factory.hpp:77] Creating layer relu6
I0122 23:02:21.989967 2968 net.cpp:86] Creating Layer relu6
I0122 23:02:21.989975 2968 net.cpp:408] relu6 <- fc6
I0122 23:02:21.989989 2968 net.cpp:369] relu6 -> fc6 (in-place)
I0122 23:02:21.990003 2968 net.cpp:124] Setting up relu6
I0122 23:02:21.990010 2968 net.cpp:131] Top shape: 10 4096 (40960)
I0122 23:02:21.990015 2968 net.cpp:139] Memory required for data: 67946040
I0122 23:02:21.990020 2968 layer_factory.hpp:77] Creating layer drop6
I0122 23:02:21.990031 2968 net.cpp:86] Creating Layer drop6
I0122 23:02:21.990036 2968 net.cpp:408] drop6 <- fc6
I0122 23:02:21.990043 2968 net.cpp:369] drop6 -> fc6 (in-place)
I0122 23:02:21.990067 2968 net.cpp:124] Setting up drop6
I0122 23:02:21.990074 2968 net.cpp:131] Top shape: 10 4096 (40960)
I0122 23:02:21.990079 2968 net.cpp:139] Memory required for data: 68109880
I0122 23:02:21.990084 2968 layer_factory.hpp:77] Creating layer fc7
I0122 23:02:21.990094 2968 net.cpp:86] Creating Layer fc7
I0122 23:02:21.990099 2968 net.cpp:408] fc7 <- fc6
I0122 23:02:21.990111 2968 net.cpp:382] fc7 -> fc7
I0122 23:02:22.008998 2968 net.cpp:124] Setting up fc7
I0122 23:02:22.009058 2968 net.cpp:131] Top shape: 10 4096 (40960)
I0122 23:02:22.009106 2968 net.cpp:139] Memory required for data: 68273720
I0122 23:02:22.009145 2968 layer_factory.hpp:77] Creating layer relu7
I0122 23:02:22.009173 2968 net.cpp:86] Creating Layer relu7
I0122 23:02:22.009187 2968 net.cpp:408] relu7 <- fc7
I0122 23:02:22.009209 2968 net.cpp:369] relu7 -> fc7 (in-place)
I0122 23:02:22.009232 2968 net.cpp:124] Setting up relu7
I0122 23:02:22.009248 2968 net.cpp:131] Top shape: 10 4096 (40960)
I0122 23:02:22.009259 2968 net.cpp:139] Memory required for data: 68437560
I0122 23:02:22.009269 2968 layer_factory.hpp:77] Creating layer drop7
I0122 23:02:22.009286 2968 net.cpp:86] Creating Layer drop7
I0122 23:02:22.009299 2968 net.cpp:408] drop7 <- fc7
I0122 23:02:22.009322 2968 net.cpp:369] drop7 -> fc7 (in-place)
I0122 23:02:22.009346 2968 net.cpp:124] Setting up drop7
I0122 23:02:22.009362 2968 net.cpp:131] Top shape: 10 4096 (40960)
I0122 23:02:22.009371 2968 net.cpp:139] Memory required for data: 68601400
I0122 23:02:22.009382 2968 layer_factory.hpp:77] Creating layer fc8
I0122 23:02:22.009399 2968 net.cpp:86] Creating Layer fc8
I0122 23:02:22.009410 2968 net.cpp:408] fc8 <- fc7
I0122 23:02:22.009428 2968 net.cpp:382] fc8 -> fc8
I0122 23:02:22.017177 2968 net.cpp:124] Setting up fc8
I0122 23:02:22.017282 2968 net.cpp:131] Top shape: 10 1000 (10000)
I0122 23:02:22.017313 2968 net.cpp:139] Memory required for data: 68641400
I0122 23:02:22.017356 2968 layer_factory.hpp:77] Creating layer prob
I0122 23:02:22.017395 2968 net.cpp:86] Creating Layer prob
I0122 23:02:22.017411 2968 net.cpp:408] prob <- fc8
I0122 23:02:22.017433 2968 net.cpp:382] prob -> prob
I0122 23:02:22.017469 2968 net.cpp:124] Setting up prob
I0122 23:02:22.017491 2968 net.cpp:131] Top shape: 10 1000 (10000)
I0122 23:02:22.017504 2968 net.cpp:139] Memory required for data: 68681400
I0122 23:02:22.017516 2968 net.cpp:202] prob does not need backward computation.
I0122 23:02:22.017554 2968 net.cpp:202] fc8 does not need backward computation.
I0122 23:02:22.017566 2968 net.cpp:202] drop7 does not need backward computation.
I0122 23:02:22.017577 2968 net.cpp:202] relu7 does not need backward computation.
I0122 23:02:22.017588 2968 net.cpp:202] fc7 does not need backward computation.
I0122 23:02:22.017598 2968 net.cpp:202] drop6 does not need backward computation.
I0122 23:02:22.017609 2968 net.cpp:202] relu6 does not need backward computation.
I0122 23:02:22.017619 2968 net.cpp:202] fc6 does not need backward computation.
I0122 23:02:22.017630 2968 net.cpp:202] pool5 does not need backward computation.
I0122 23:02:22.017642 2968 net.cpp:202] relu5 does not need backward computation.
I0122 23:02:22.017652 2968 net.cpp:202] conv5 does not need backward computation.
I0122 23:02:22.017663 2968 net.cpp:202] relu4 does not need backward computation.
I0122 23:02:22.017674 2968 net.cpp:202] conv4 does not need backward computation.
I0122 23:02:22.017685 2968 net.cpp:202] relu3 does not need backward computation.
I0122 23:02:22.017696 2968 net.cpp:202] conv3 does not need backward computation.
I0122 23:02:22.017707 2968 net.cpp:202] norm2 does not need backward computation.
I0122 23:02:22.017720 2968 net.cpp:202] pool2 does not need backward computation.
I0122 23:02:22.017734 2968 net.cpp:202] relu2 does not need backward computation.
I0122 23:02:22.017746 2968 net.cpp:202] conv2 does not need backward computation.
I0122 23:02:22.017757 2968 net.cpp:202] norm1 does not need backward computation.
I0122 23:02:22.017770 2968 net.cpp:202] pool1 does not need backward computation.
I0122 23:02:22.017783 2968 net.cpp:202] relu1 does not need backward computation.
I0122 23:02:22.017796 2968 net.cpp:202] conv1 does not need backward computation.
I0122 23:02:22.017809 2968 net.cpp:202] input does not need backward computation.
I0122 23:02:22.017819 2968 net.cpp:244] This network produces output prob
I0122 23:02:22.017868 2968 net.cpp:257] Network initialization done.
I0122 23:02:22.196004 2968 upgrade_proto.cpp:44] Attempting to upgrade input file specified using deprecated transformation parameters: /home/relaybot/Rob_Soft/caffe/src/ros_caffe/data/bvlc_reference_caffenet.caffemodel
I0122 23:02:22.196061 2968 upgrade_proto.cpp:47] Successfully upgraded file specified using deprecated data transformation parameters.
W0122 23:02:22.196069 2968 upgrade_proto.cpp:49] Note that future Caffe releases will only support transform_param messages for transformation fields.
I0122 23:02:22.196074 2968 upgrade_proto.cpp:53] Attempting to upgrade input file specified using deprecated V1LayerParameter: /home/relaybot/Rob_Soft/caffe/src/ros_caffe/data/bvlc_reference_caffenet.caffemodel
I0122 23:02:22.506147 2968 upgrade_proto.cpp:61] Successfully upgraded file specified using deprecated V1LayerParameter
I0122 23:02:22.507925 2968 net.cpp:746] Ignoring source layer data
I0122 23:02:22.597734 2968 net.cpp:746] Ignoring source layer loss
W0122 23:02:22.716584 2968 net.hpp:41] DEPRECATED: ForwardPrefilled() will be removed in a future version. Use Forward().
Test default image under /data/cat.jpg
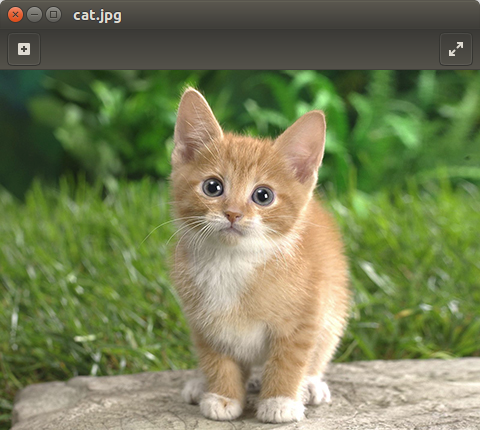
0.3134 - "n02123045 tabby, tabby cat"
0.2380 - "n02123159 tiger cat"
0.1235 - "n02124075 Egyptian cat"
0.1003 - "n02119022 red fox, Vulpes vulpes"
0.0715 - "n02127052 lynx, catamount"
W0122 23:07:35.308277 2968 net.hpp:41] DEPRECATED: ForwardPrefilled() will be removed in a future version. Use Forward().
W0122 23:12:52.805382 2968 net.hpp:41] DEPRECATED: ForwardPrefilled() will be removed in a future version. Use Forward().
$ rostopic list
/camera/rgb/image_raw
/camera_info
/image_raw
/image_raw/compressed
/image_raw/compressed/parameter_descriptions
/image_raw/compressed/parameter_updates
/image_raw/compressedDepth
/image_raw/compressedDepth/parameter_descriptions
/image_raw/compressedDepth/parameter_updates
/image_raw/theora
/image_raw/theora/parameter_descriptions
/image_raw/theora/parameter_updates
/rosout
/rosout_agg
$ rostopic echo /caffe_ret
---
data: [0.557911 - n04286575 spotlight, spot]
[0.115966 - n03729826 matchstick]
[0.0737537 - n02948072 candle, taper, wax light]
[0.040883 - n09472597 volcano]
[0.028961 - n03666591 lighter, light, igniter, ignitor]
---
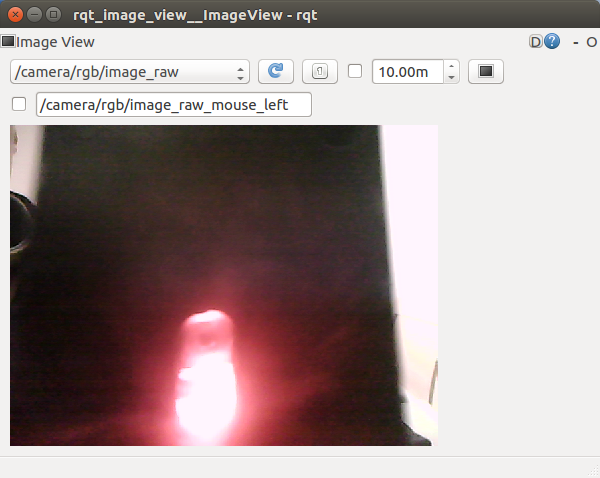
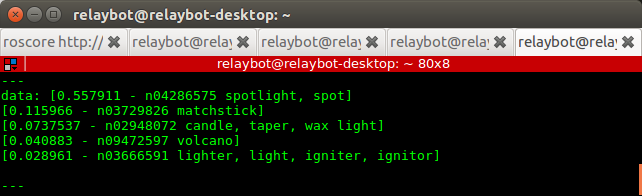
$ rosrun rqt_graph rqt_graph
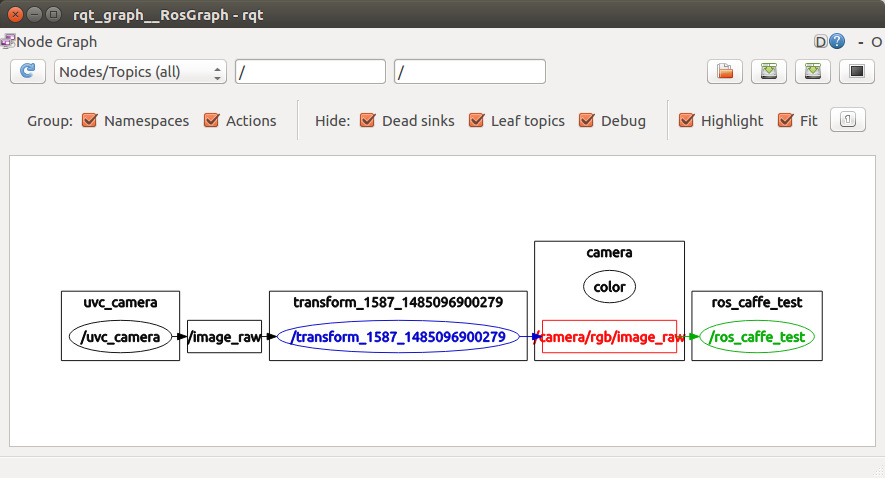
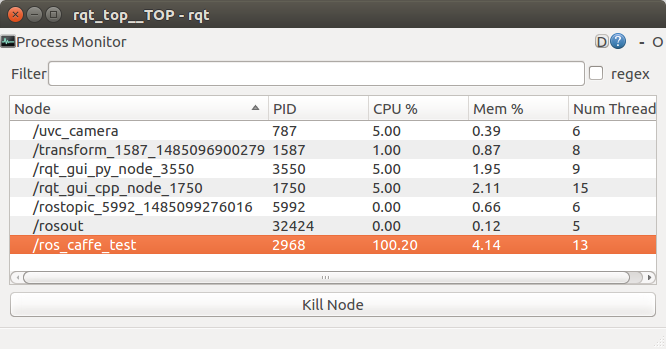
-End-
本文内容由网友自发贡献,版权归原作者所有,本站不承担相应法律责任。如您发现有涉嫌抄袭侵权的内容,请联系:hwhale#tublm.com(使用前将#替换为@)