MapReduce阶段分为map,shuffle,reduce。
map进行数据的映射,就是数据结构的转换,shuffle是一种内存缓冲,同时对map后的数据分区、排序。reduce则是最后的聚合。
此文探讨map阶段的主要工作。
代码介绍
我们还是准备word count的代码:
maper:
public class WordCountMapper extends Mapper<LongWritable, Text, Text, LongWritable> {
Text mapOutKey = new Text();
LongWritable mapOutValue = new LongWritable(1);
@Override
protected void map(LongWritable key, Text value, Context context) throws IOException, InterruptedException {
String line = value.toString();
String[] words = line.split(" ");
for (String word : words) {
mapOutKey.set(word);
context.write(mapOutKey, mapOutValue);
}
}
}
reducer:
public class WordCountReducer extends Reducer<Text, LongWritable, Text, LongWritable> {
LongWritable reduceOutValue = new LongWritable();
@Override
protected void reduce(Text key, Iterable<LongWritable> values, Context context) throws IOException, InterruptedException {
Long sum = 0L;
for (LongWritable value : values) {
sum += value.get();
}
reduceOutValue.set(sum);
context.write(key, reduceOutValue);
}
}
partitioner:
public class WordCountPartitioner extends Partitioner<Text, LongWritable> {
@Override
public int getPartition(Text text, LongWritable longWritable, int numPartitions) {
int partition = 0;
String word = text.toString();
if("I".equalsIgnoreCase(word)){
partition = 1;
}
return partition;
}
}
driver:
public class WordCountDriver implements Tool {
private Configuration configuration = null;
static {
try {
System.setProperty("hadoop.home.dir", "D:\\hadoop\\hadoop-3.1.0");
System.load("D:\\hadoop\\hadoop-3.1.0\\bin\\hadoop.dll");
} catch (UnsatisfiedLinkError e) {
System.err.println("Native code library failed to load.\n" + e);
System.exit(1);
}
}
@Override
public int run(String[] args) throws Exception {
Job job = Job.getInstance(this.configuration);
job.setJarByClass(WordCountDriver.class);
job.setMapperClass(WordCountMapper.class);
job.setReducerClass(WordCountReducer.class);
job.setMapOutputKeyClass(Text.class);
job.setMapOutputValueClass(LongWritable.class);
job.setOutputKeyClass(Text.class);
job.setOutputValueClass(LongWritable.class);
job.setPartitionerClass(WordCountPartitioner.class);
job.setNumReduceTasks(2);
FileInputFormat.setInputPaths(job, new Path("D:\\hadoop\\input\\blogInput"));
FileOutputFormat.setOutputPath(job, new Path("D:\\hadoop\\output\\blogOutput"));
boolean result = job.waitForCompletion(true);
return result ? 1 : 0;
}
@Override
public void setConf(Configuration conf) {
configuration = conf;
}
@Override
public Configuration getConf() {
return configuration;
}
public static void main(String[] args) {
try {
ToolRunner.run(new Configuration(), new WordCountDriver(), args);
} catch (Exception e) {
e.printStackTrace();
}
}
}
input:
I like java
I like scala
I like python
I hate hadoop
使用自定的partitioner是因为我们想看到分区的逻辑。
如果你没有自己的partitioner,一个分区的话(默认就是一个reduceTask和一个partition):
框架给你自己new一个匿名类,返回的partition只有0,即一个分区:
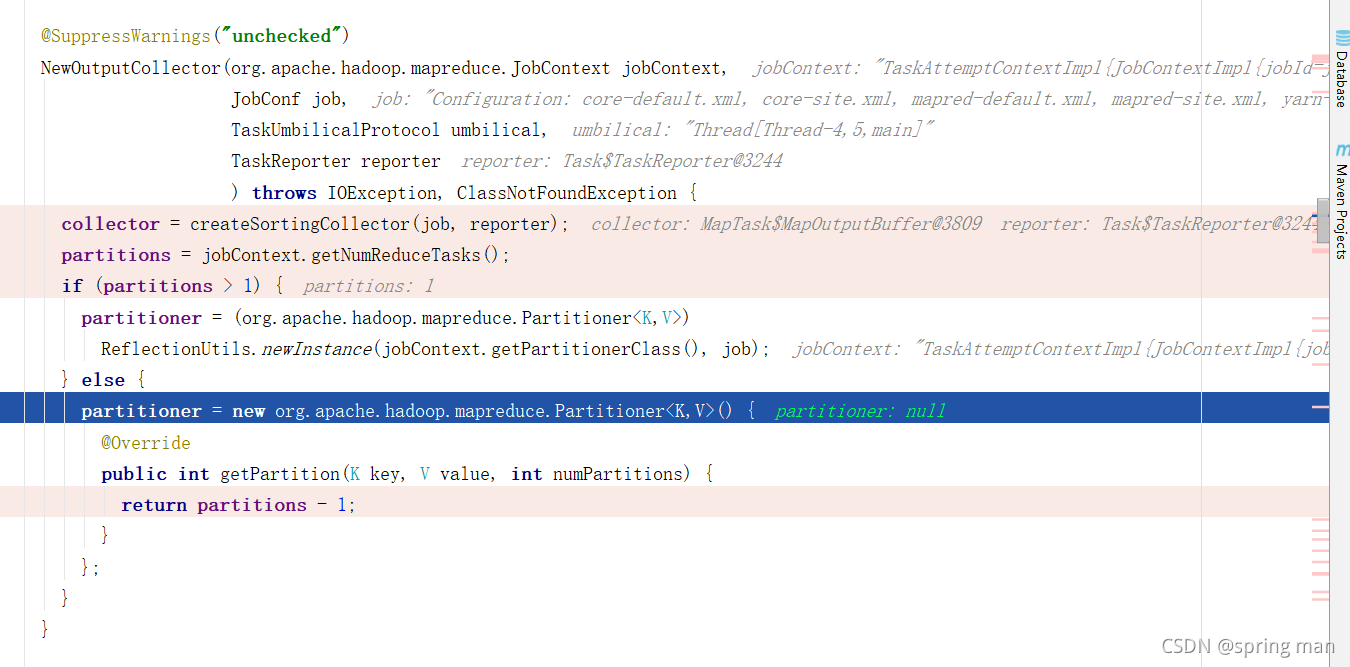
如果你没有自己的partitioner,但是reduceTask的数量超过了一个,比如这样:
job.setNumReduceTasks(2);
此时默认用的是HashPartitioner
:
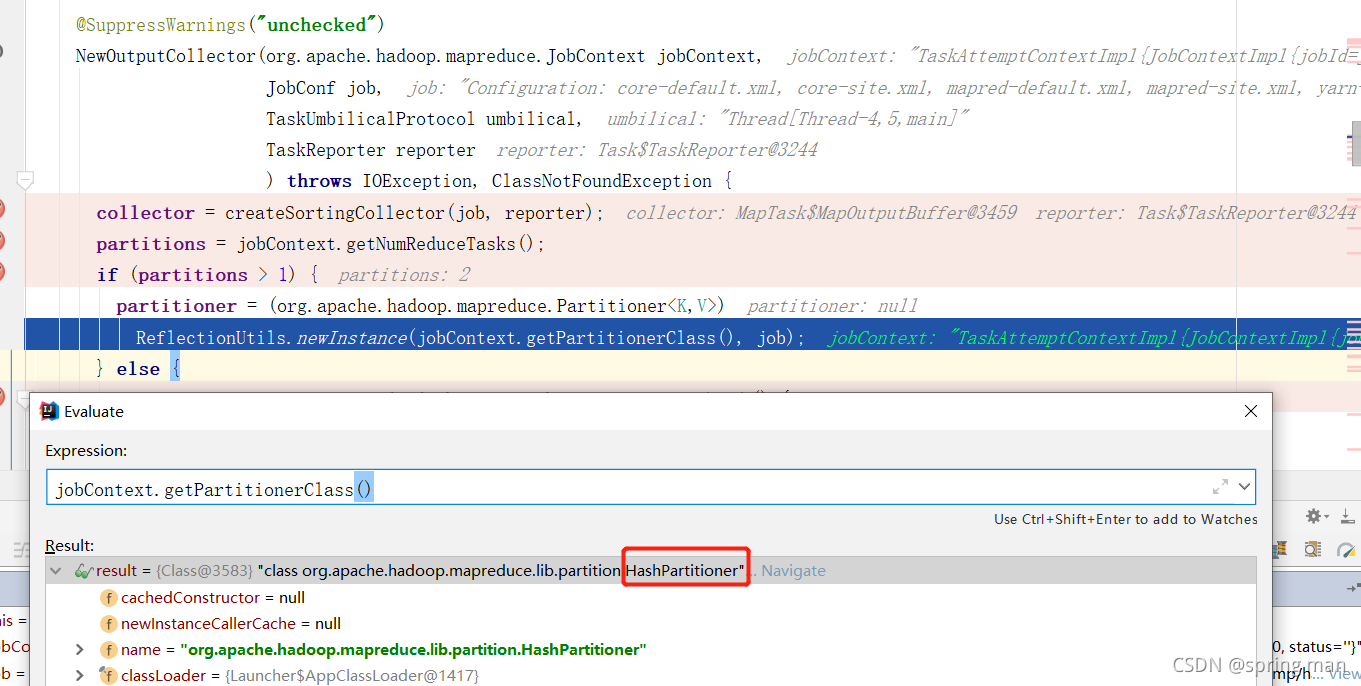
split
hdfs中需要分块,那是实际的将文件切分存储到磁盘。
mapreduce的map会涉及到切片(split),那是一种逻辑的分割,就是代码层面的,每个切片开一个mapTask来处理。
首先明确我们的环境,因为使用的是本地运行,所以任务是提交到本地而非yarn:
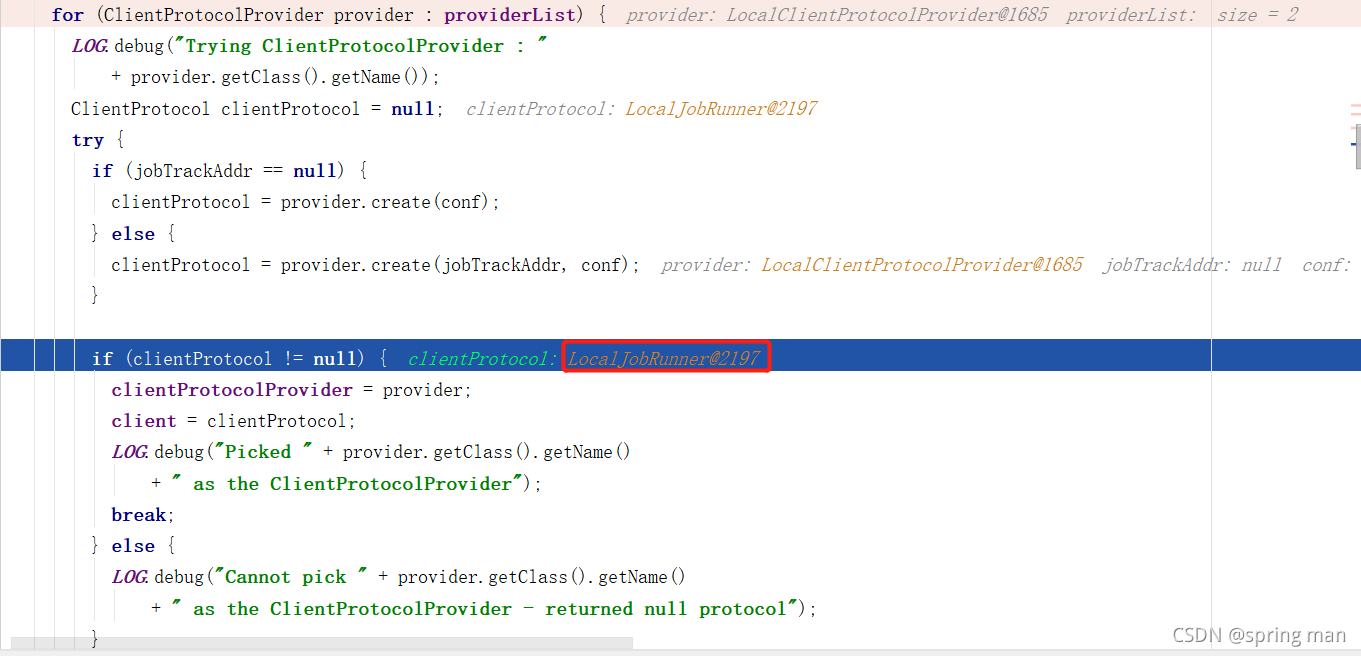
由于是本地,所以也无需提交jar包,yarn的话就需要了:
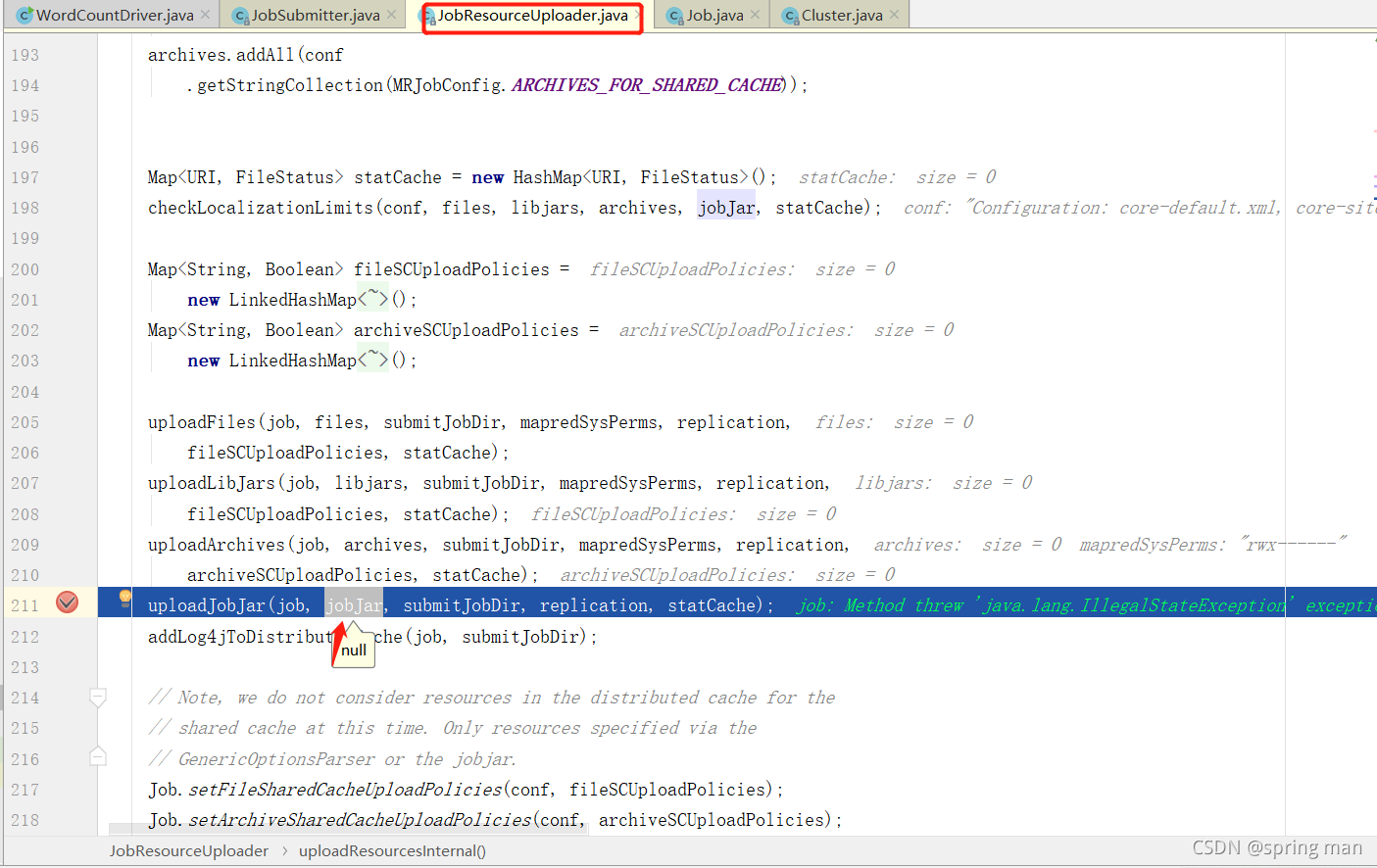
接下来开始切片,它会将切片文件放在本地临时文件夹:
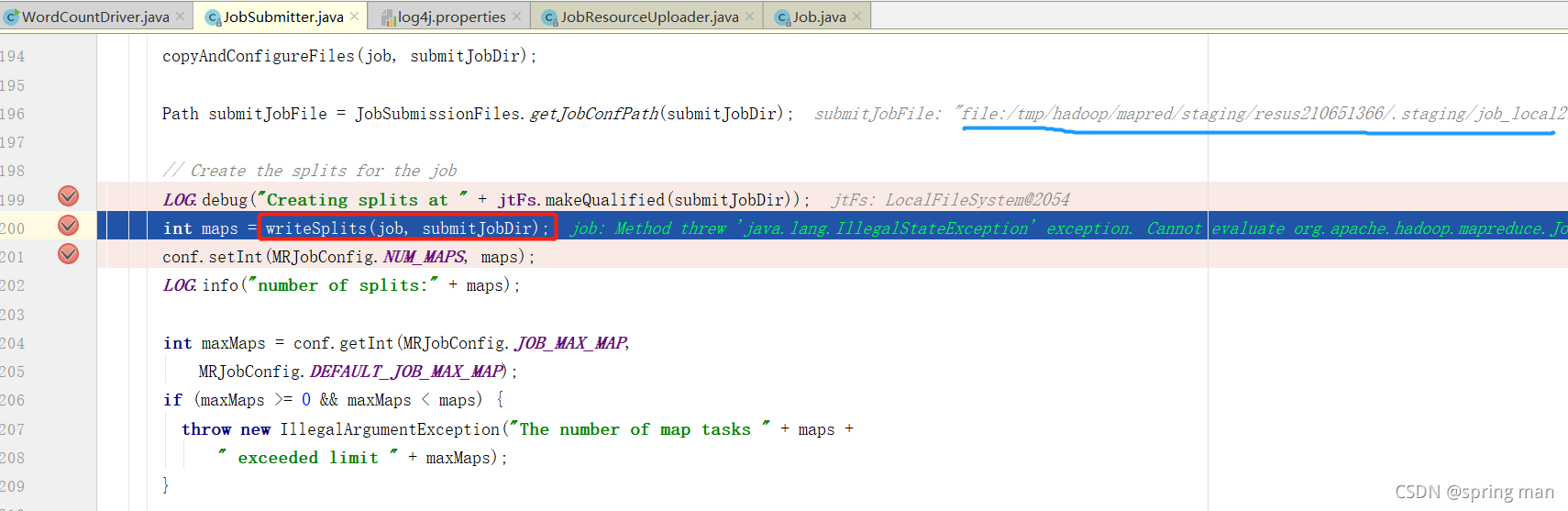
切片大小由三个值决定,块大小,一个最大值,一个最小值。
本地模式下块大小是32MB,因为他认为你本地资源不足。
最小值我这里是1,最大值是Long的最大值,你可以去配。
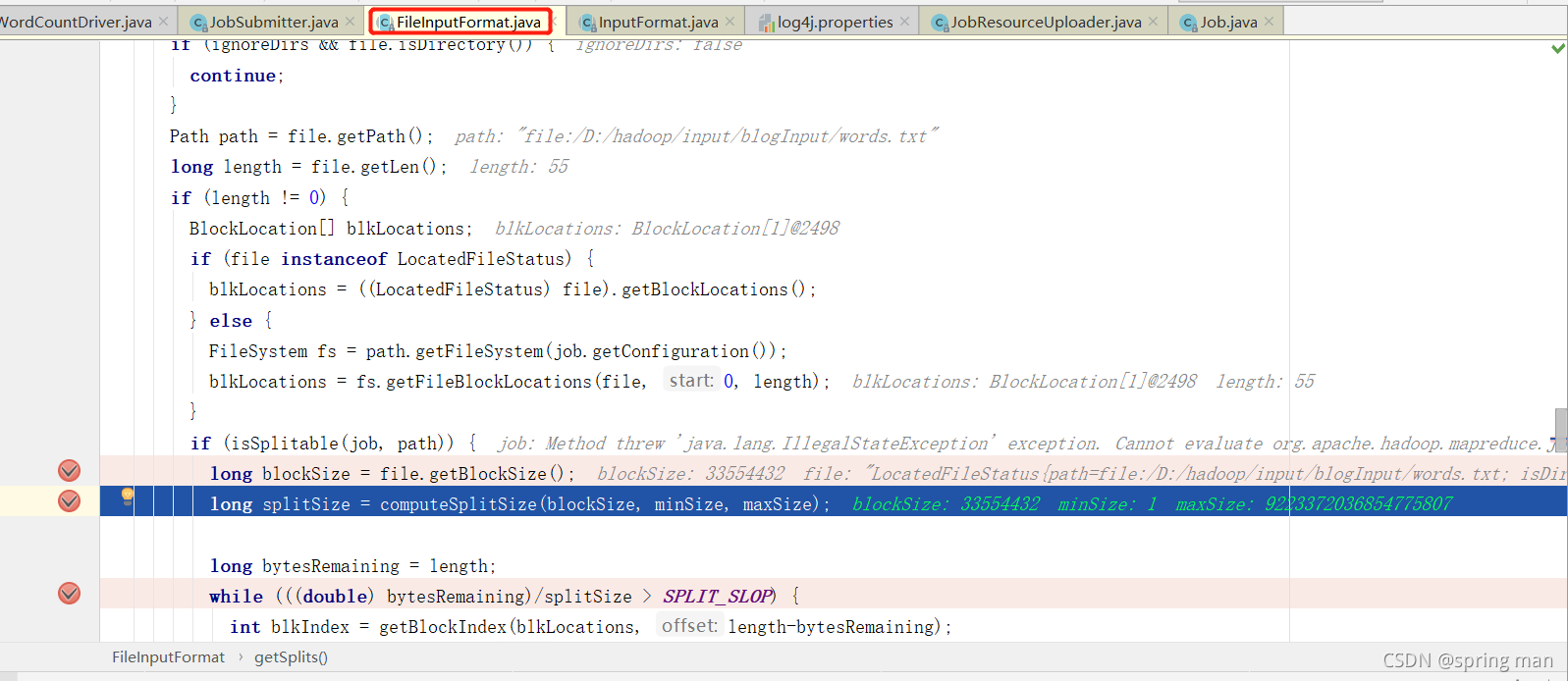

最后算出来切片大小就是块大小。
或者我们可以说,默认情况下切片大小就是块大小。
但这里又不是简单按照32MB进行切的:
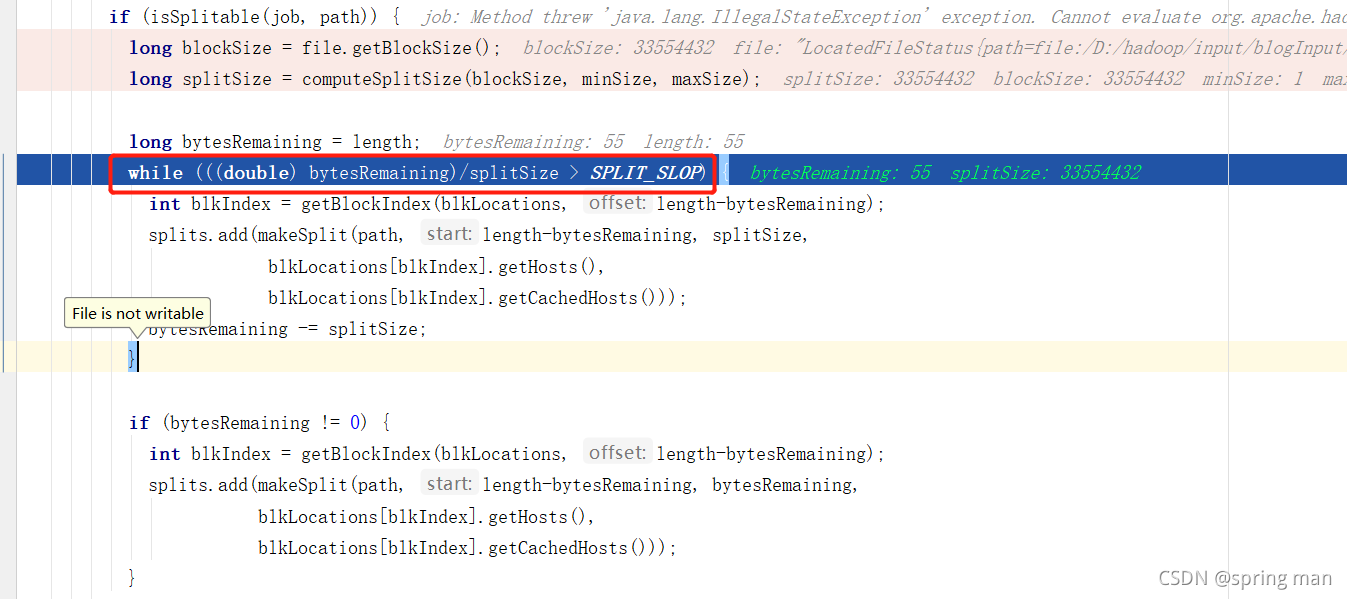
SPLIT_SLOP
是1.1,也就是说,如果文件大小是40MB,没问题,切成两片,一片32MB,一片8MB;但如果文件大小是32.1MB,它去除以32没有大于1.1,那就还是一片。
其实这就防止了小文件的产生,32.1MB还切成两片,给0.1MB开启一个mapTask,耗费1G的内存,任务启动时间大于任务计算时间,这是不合理的。
最后将切片信息写到本地:
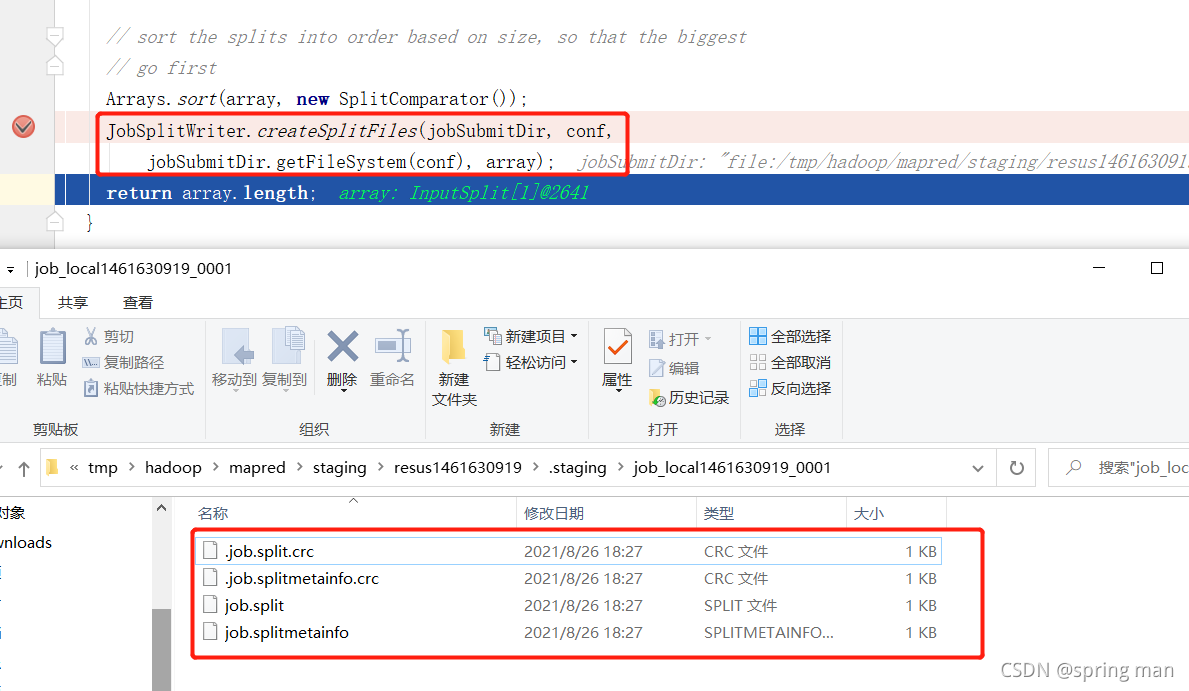
我们再返回到map的处理流程:
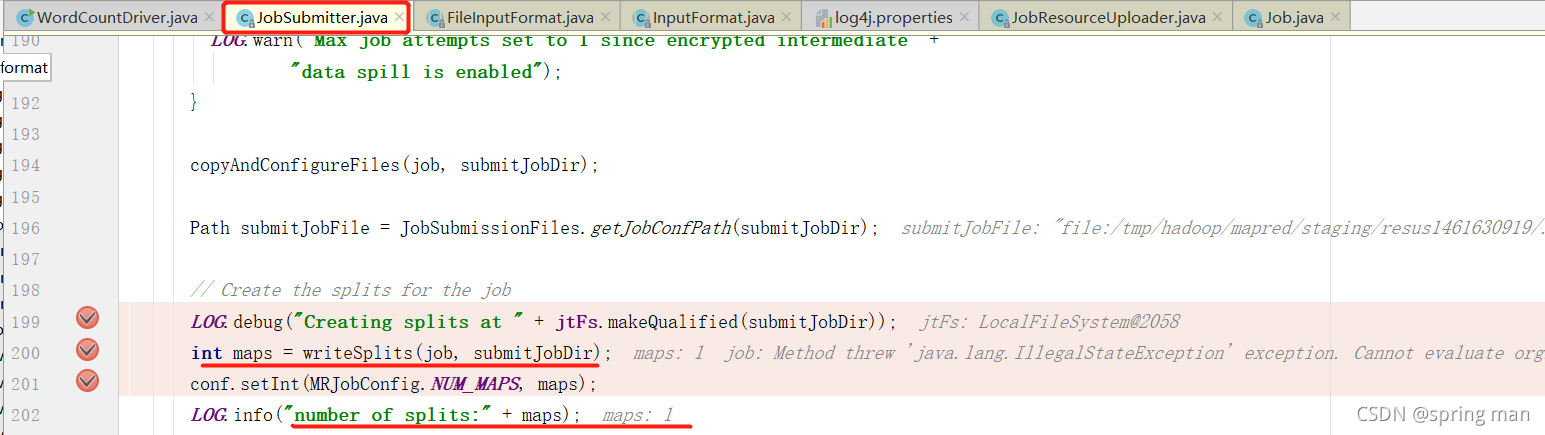
从这里也可以看到切片的数量影响mapTask的数量。
接着我们将提交配置信息。
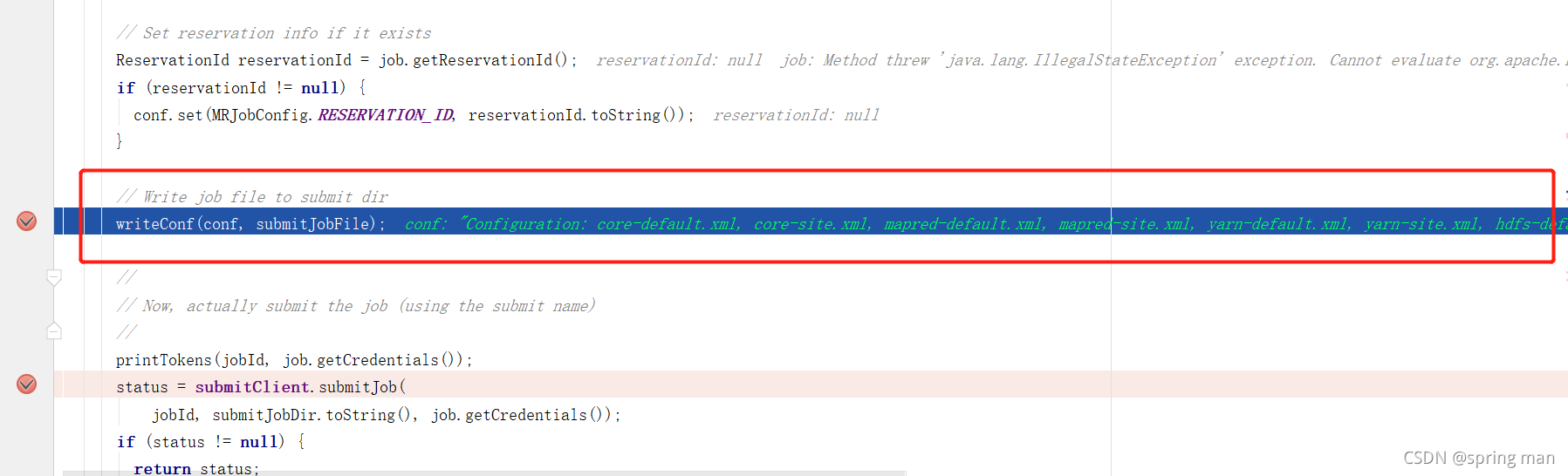
启动mapTask
当我们new job的时候调用
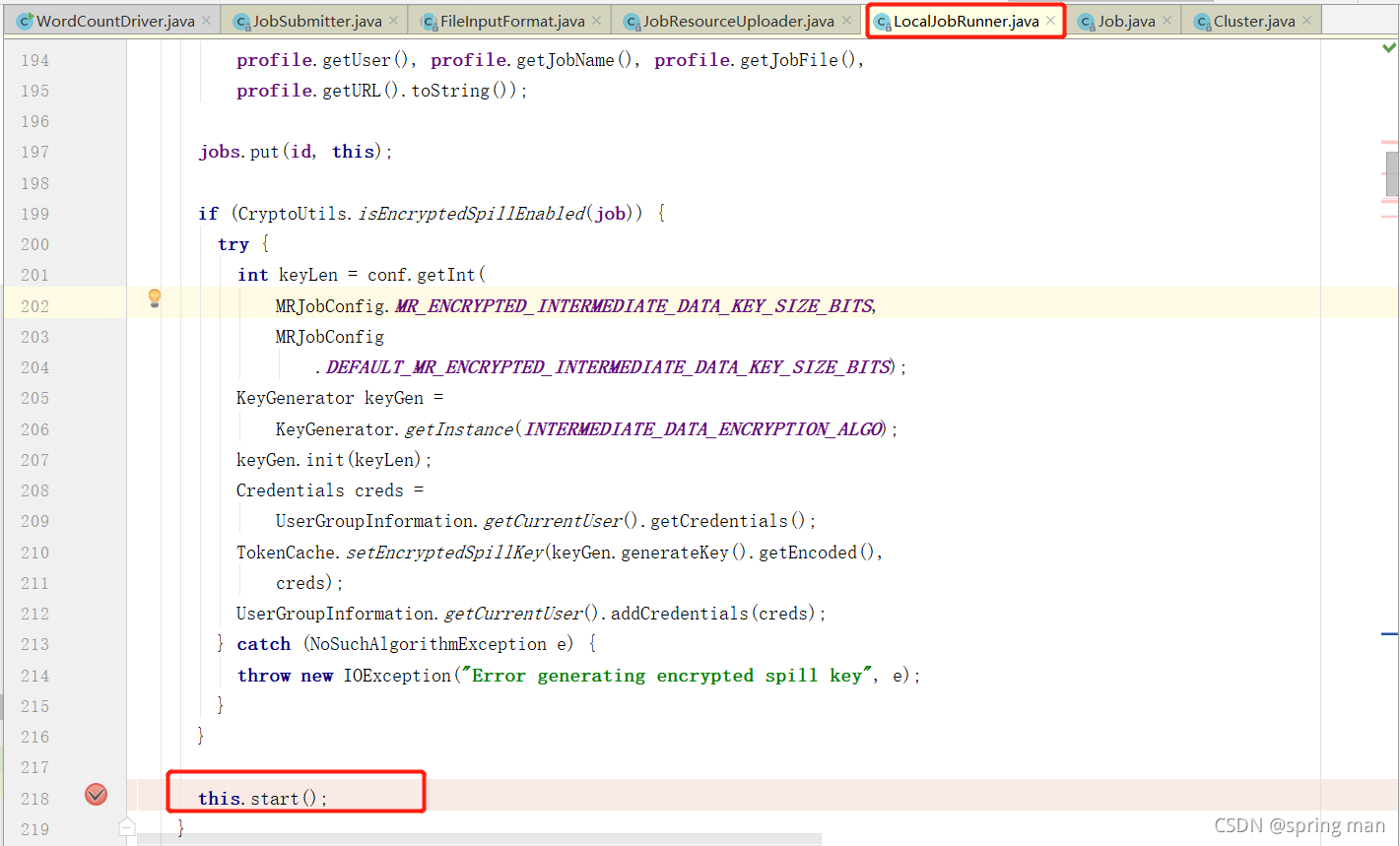
一个任务就开启了。
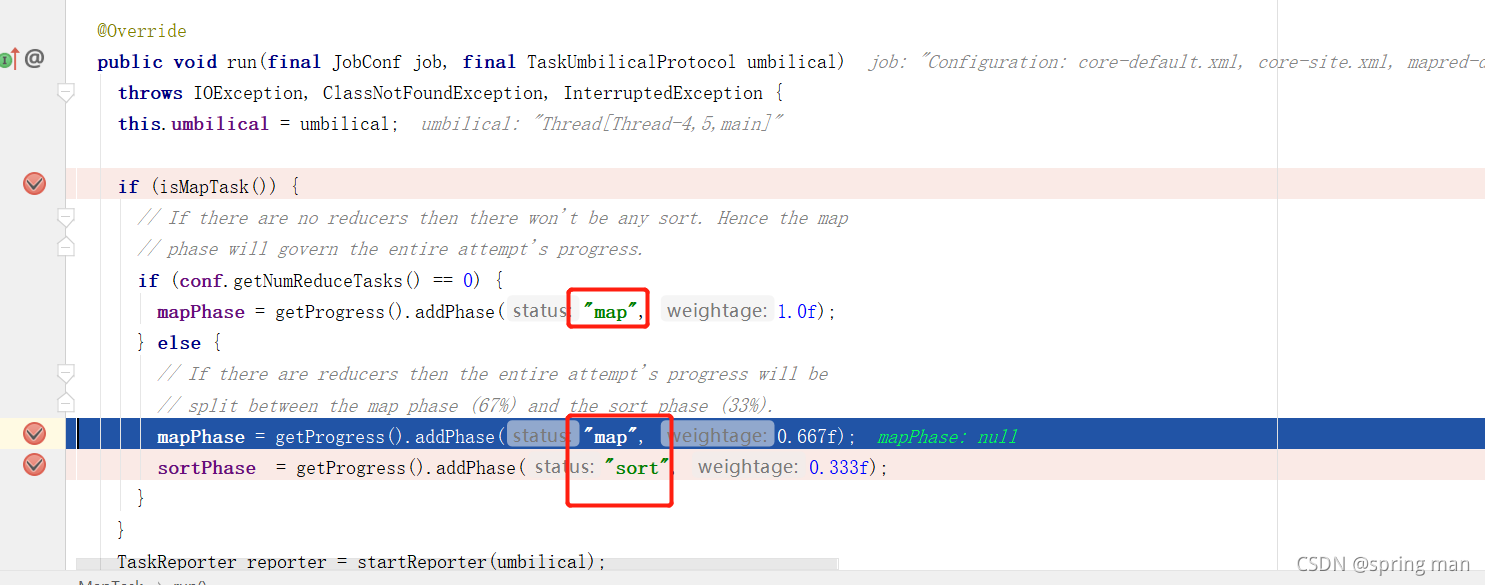
如果没有reduce阶段,那么直接map即可。
如果有reduce阶段,那么先map再sort。
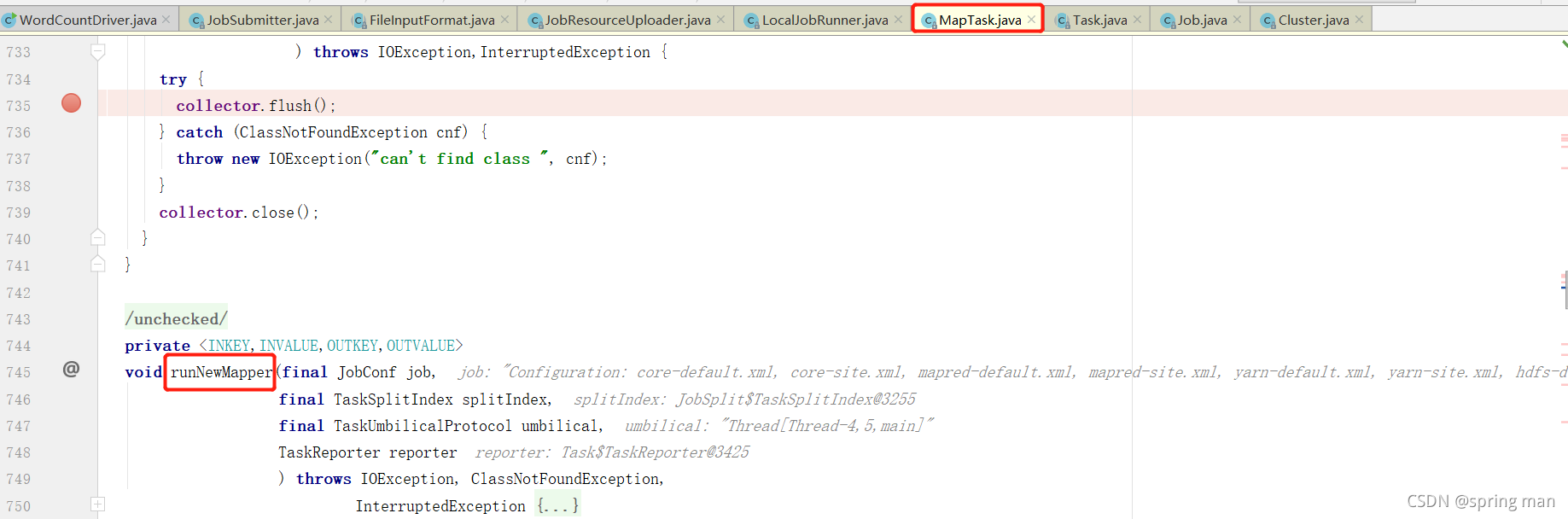
这个方法将真正启动map。
首先,我们需要一个采集器,接受map端输出的数据:
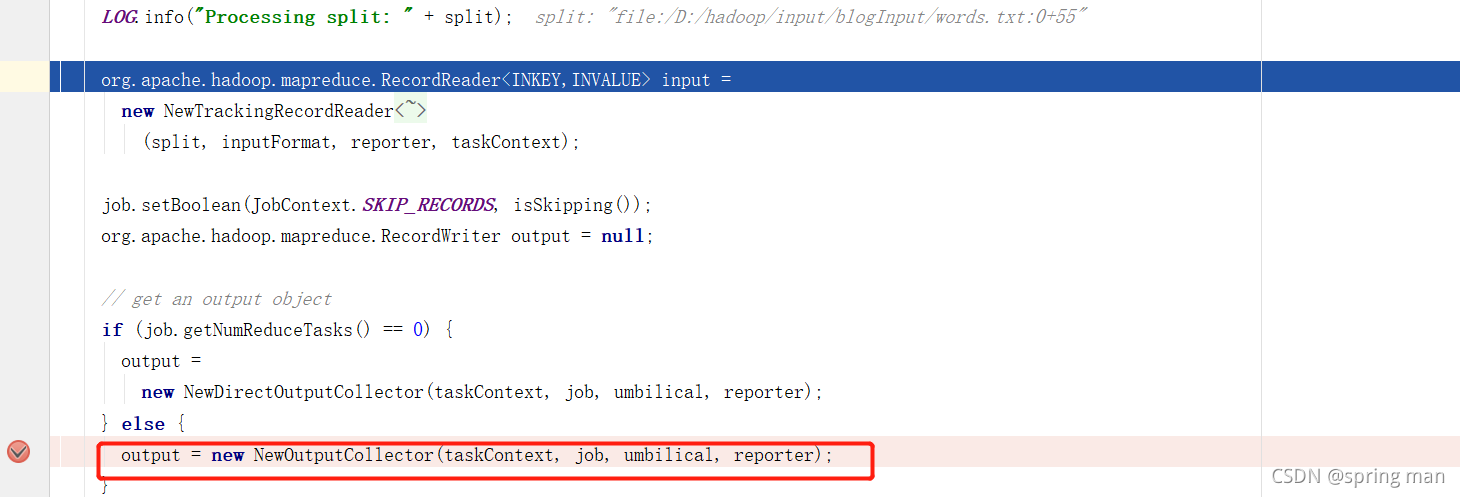
初始化采集器时,我们将注意到几个重要的参数,它们将构建后来的缓冲区和排序和溢写:
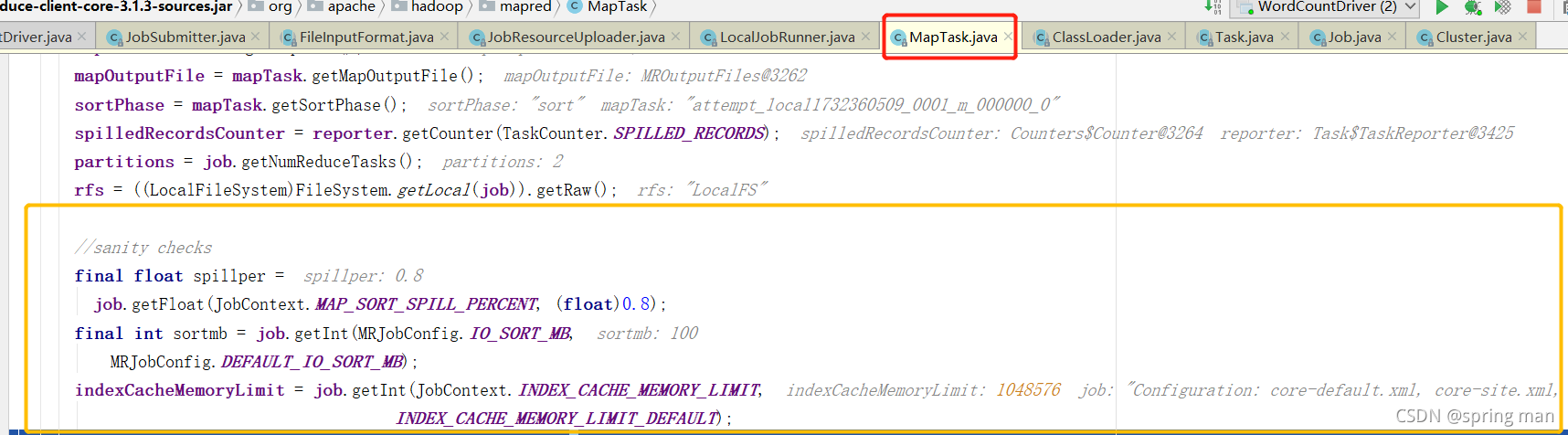
缓冲区的默认大小是100MB。
spillper0.8是溢写的阈值,就是内存到80MB了,那就把数据写到磁盘上,这样可以保证读取数据和写出数据都正常进行。
索引的缓存上限是1MB,如果索引在内存中大于1MB了,那就写到磁盘。
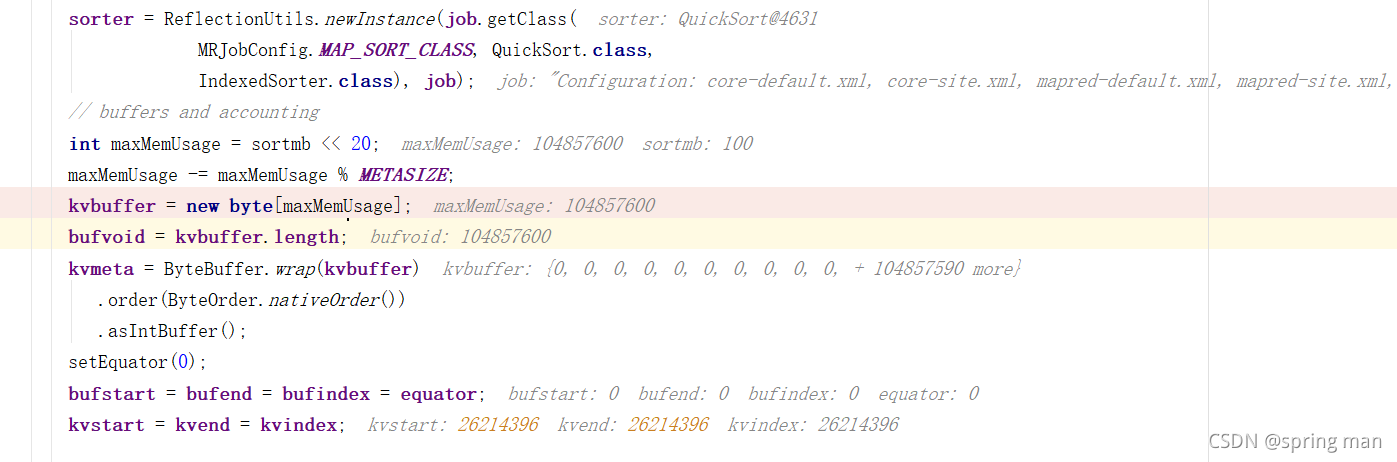
缓冲区的数据溢写到磁盘之前要进行排序,排序的算法是快排。
缓冲区叫做kvbuffer,100MB。
存索引的叫kvmeta,这是一个IntBuffer,它的大小其实也是100MB,只不过1的int占4个字节,所以它的capacity就是100MB/4。
bufstart = bufend = bufindex = equator;
都是0,这是和真实数据相关的。
kvstart = kvend = kvindex;
都是26214396,它是存元数据的kvmeta的一个位置,最后将不断往前跳4个位置来存储元数据。
bufferRemaining
默认就是80MB(图上没显示)。
初始化采集器的时候同时溢写线程也开启了:
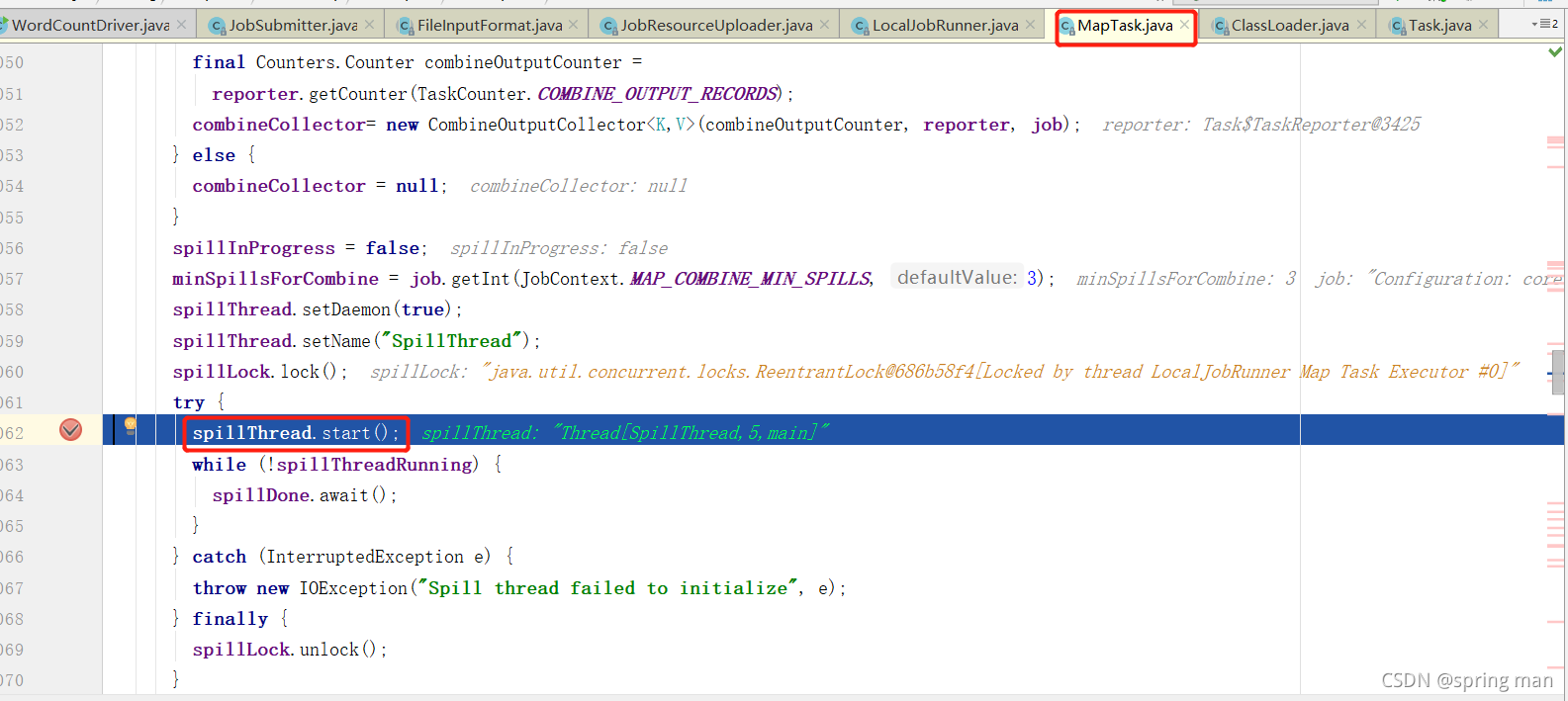
map的初始化工作算是完成了。接着就要走进我们程序的map处理逻辑了:
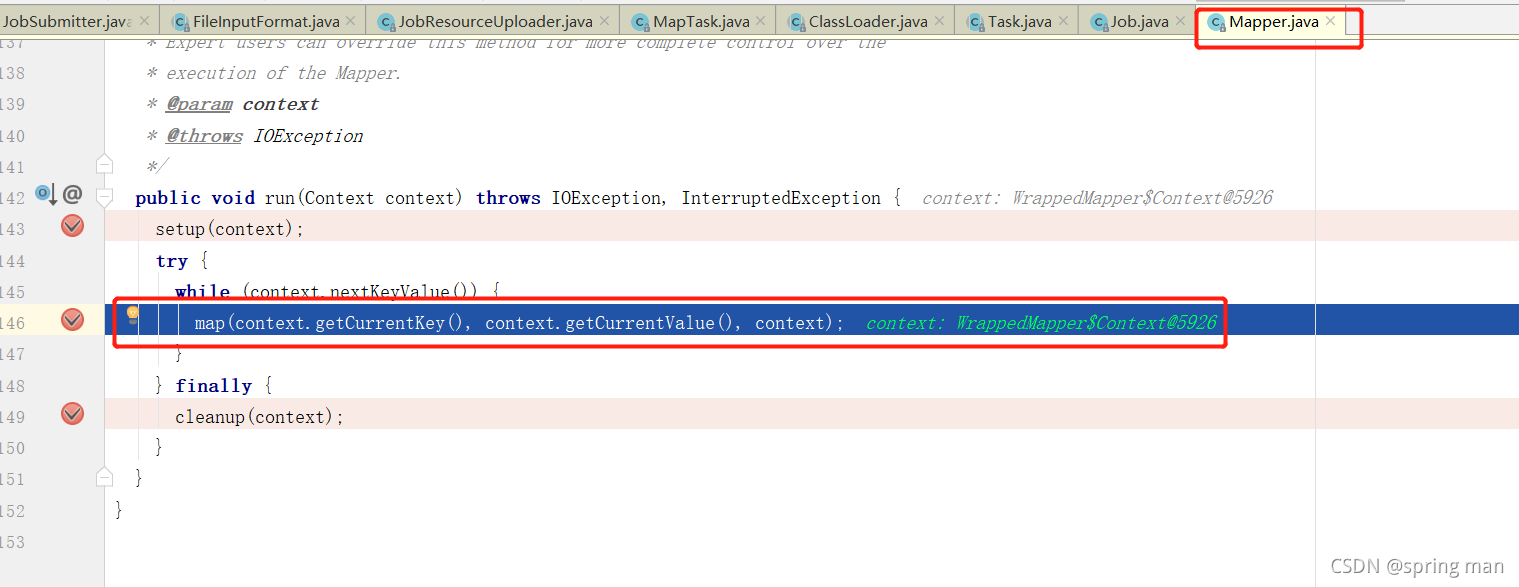
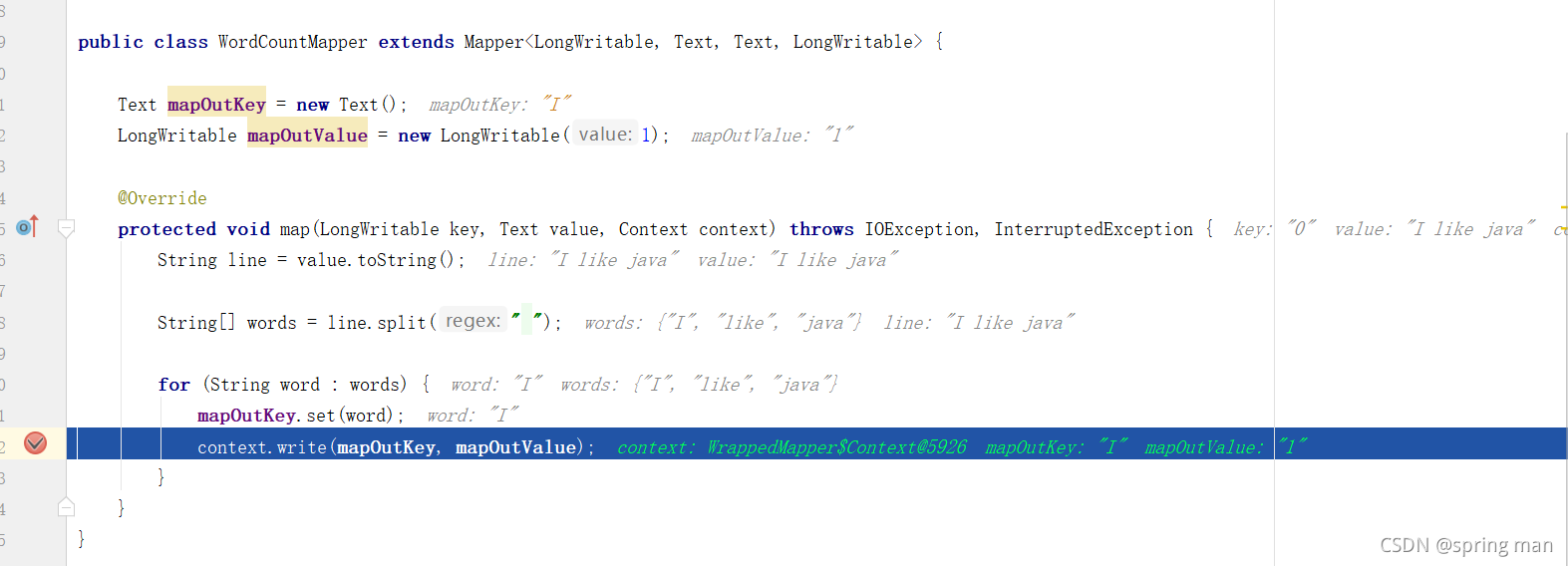
读取的第一行是I like java
。
我们将key为I,value为1的数据往外写,写到哪里去呢?就是初始化好的缓冲区和存元数据的IntBuffer。我们把下个阶段叫shuffle。
本文内容由网友自发贡献,版权归原作者所有,本站不承担相应法律责任。如您发现有涉嫌抄袭侵权的内容,请联系:hwhale#tublm.com(使用前将#替换为@)