目录
一、解决报错ModuleNotFoundError: No module named ‘tensorflow.examples
二、解决报错ModuleNotFoundError: No module named ‘tensorflow.contrib‘
三、安装onnx报错assert CMAKE, ‘Could not find “cmake“ executable!‘
四、ImportError: cannot import name 'builder' from 'google.protobuf.internal'
五、解决ModuleNotFoundError: No module named 'sklearn'
六、解决AttributeError: module ‘torch._C‘ has no attribute ‘_cuda_setDevice‘
七、解决ImportError: Missing optional dependency 'pytables'. Use pip or conda to install pytables.
八、解决AttributeError: module ‘distutils’ has no attribute ‘version’.
一、解决报错ModuleNotFoundError: No module named ‘tensorflow.examples
注意:MNIST数据集下载完成后不要解压,直接放入mnist_data文件夹下读取即可。
问题:我在用tensorflow做mnist数据集案例,报错了。

原因:tensorflow中没有examples。
解决方法:(1)首先找到对应tensorflow的文件,我的是在D:\python3\Lib\site-packages\tensorflow(python的安装目录),进入tensorflow文件夹,发现没有examples文件夹。
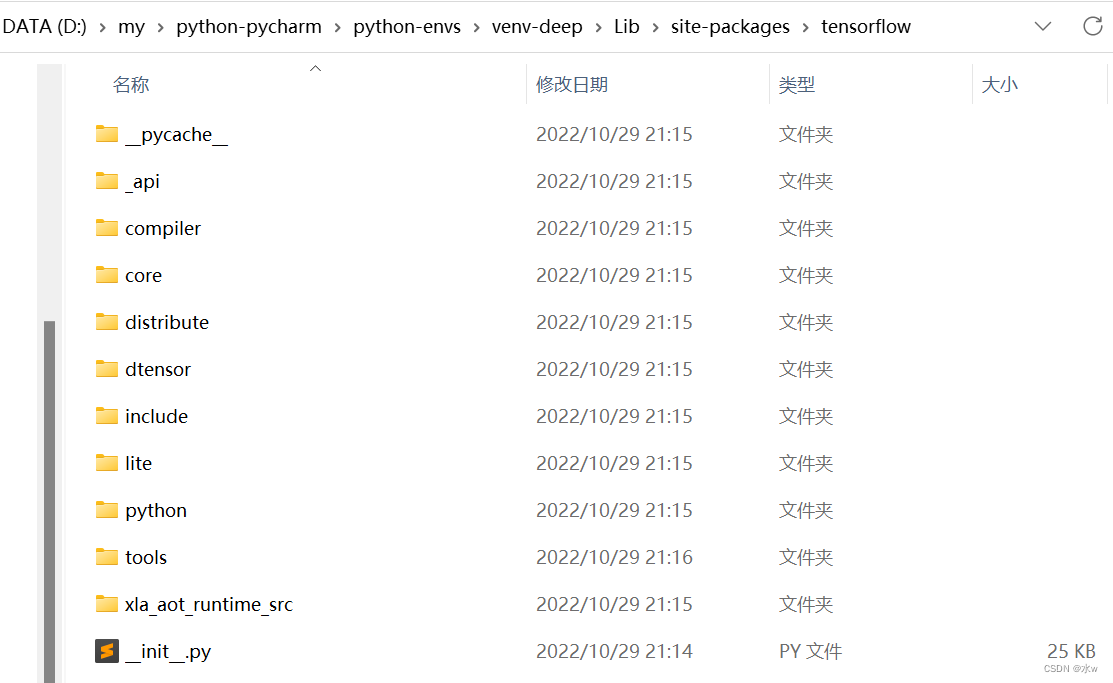
我们可以进入github下载:mirrors / tensorflow / tensorflow · GitCode。
(2)下载完成后将\tensorflow-master\tensorflow\目录下的examples文件夹复制到本地tensorflow文件夹中,然后在重新运行代码即可。
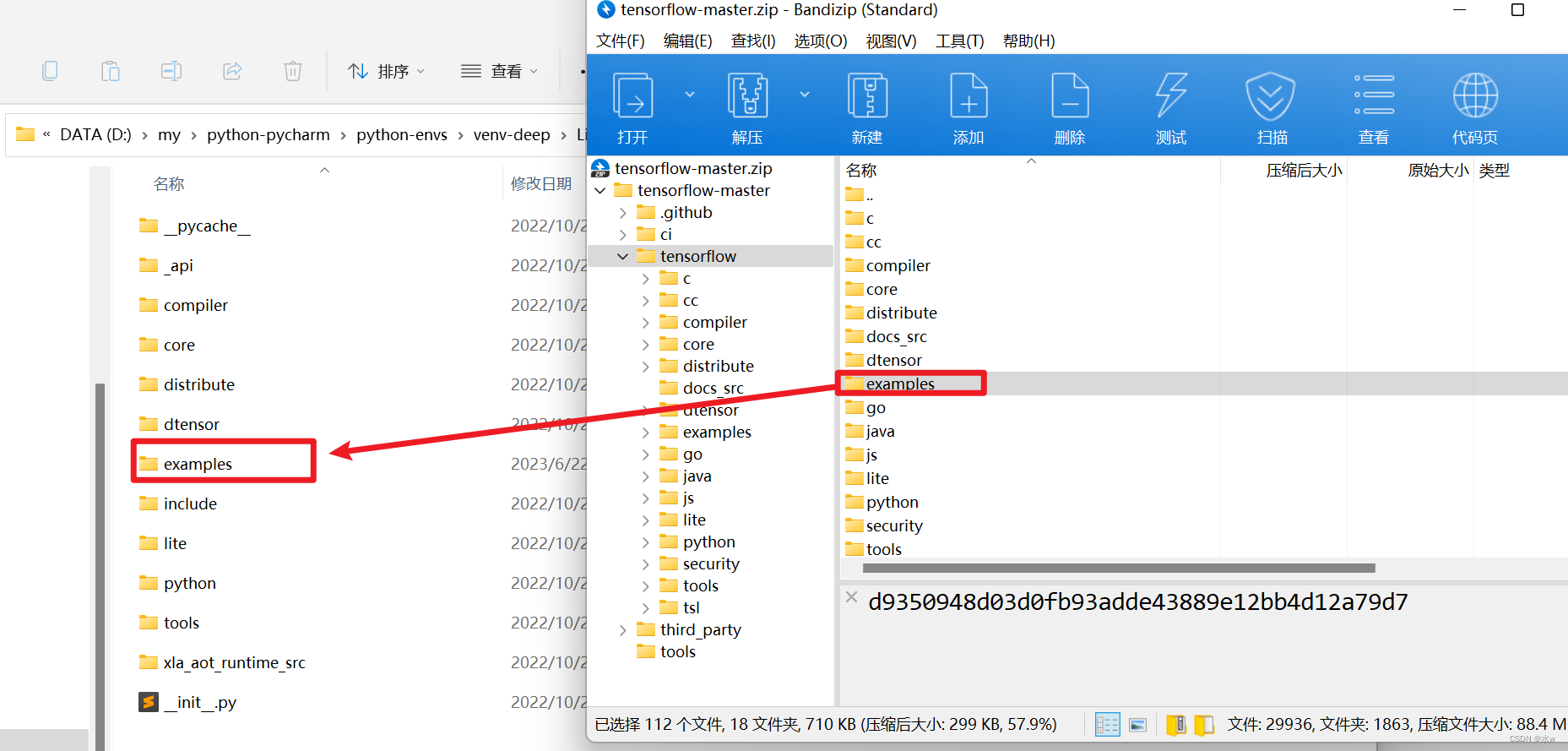
(3)之后发现还是没能解决问题,发现examples中缺少tutorials文件夹。在官方的github中没发现这个文件,在其他博主那里下载到了该文件。

下载地址: 百度网盘 请输入提取码
提取码:cxy7
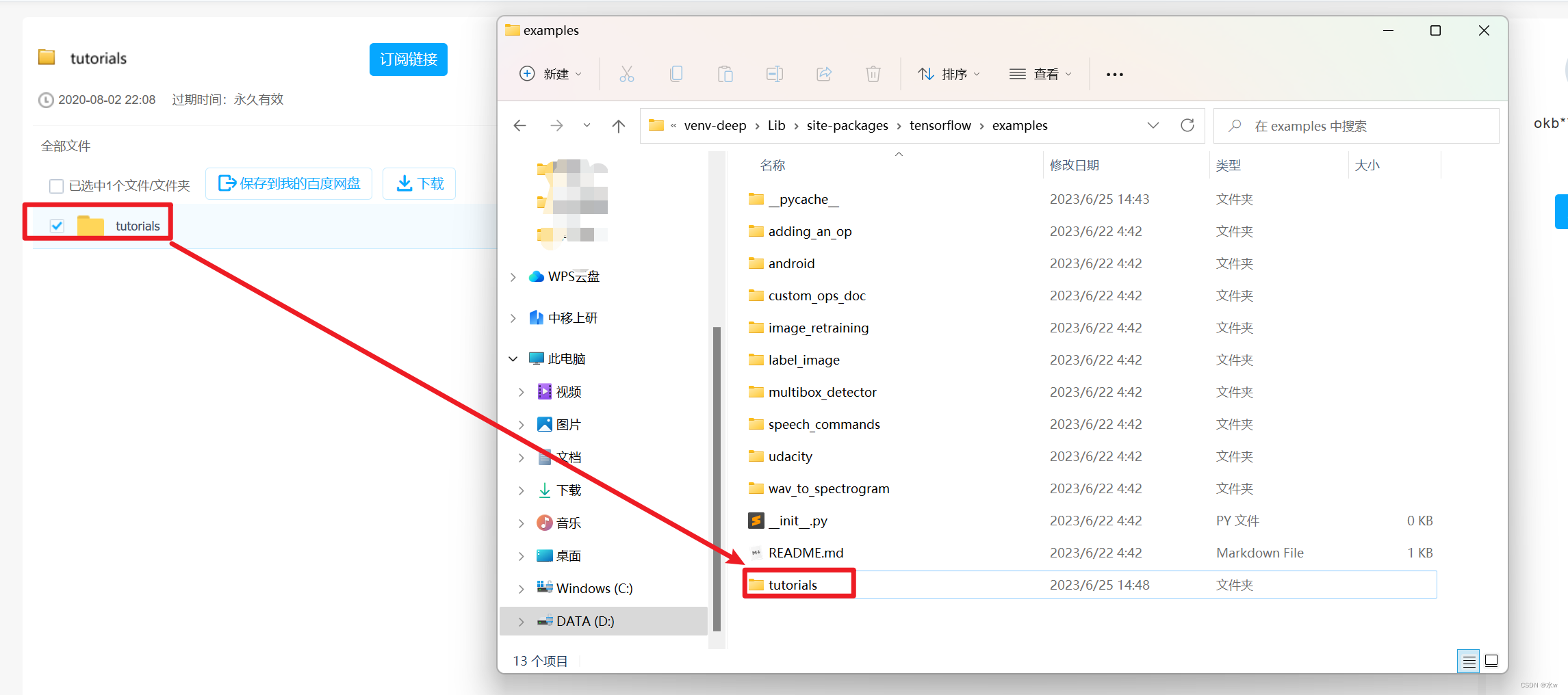
(4)但是依旧没有解决问题…
前面博主使用的应该是tf1.0的版本。参考其他博主的方法解决了问题。
- 在工程下新建一个input_data.py文件,将tutorials文件夹下mnist中的input_data.py的内容复制到该文件中,
- 再在主文件中import input_data一下。
input_data.py文件内容放在下面,需要的自取。
# Copyright 2016 The TensorFlow Authors. All Rights Reserved.
#
# Licensed under the Apache License, Version 2.0 (the "License");
# you may not use this file except in compliance with the License.
# You may obtain a copy of the License at
#
# http://www.apache.org/licenses/LICENSE-2.0
#
# Unless required by applicable law or agreed to in writing, software
# distributed under the License is distributed on an "AS IS" BASIS,
# WITHOUT WARRANTIES OR CONDITIONS OF ANY KIND, either express or implied.
# See the License for the specific language governing permissions and
# limitations under the License.
# ==============================================================================
"""Functions for downloading and reading MNIST data (deprecated).
This module and all its submodules are deprecated.
"""
from __future__ import absolute_import
from __future__ import division
from __future__ import print_function
import collections
import gzip
import os
import numpy
from six.moves import urllib
from six.moves import xrange # pylint: disable=redefined-builtin
from tensorflow.python.framework import dtypes
from tensorflow.python.framework import random_seed
from tensorflow.python.platform import gfile
from tensorflow.python.util.deprecation import deprecated
_Datasets = collections.namedtuple('_Datasets', ['train', 'validation', 'test'])
# CVDF mirror of http://yann.lecun.com/exdb/mnist/
DEFAULT_SOURCE_URL = 'https://storage.googleapis.com/cvdf-datasets/mnist/'
def _read32(bytestream):
dt = numpy.dtype(numpy.uint32).newbyteorder('>')
return numpy.frombuffer(bytestream.read(4), dtype=dt)[0]
@deprecated(None, 'Please use tf.data to implement this functionality.')
def _extract_images(f):
"""Extract the images into a 4D uint8 numpy array [index, y, x, depth].
Args:
f: A file object that can be passed into a gzip reader.
Returns:
data: A 4D uint8 numpy array [index, y, x, depth].
Raises:
ValueError: If the bytestream does not start with 2051.
"""
print('Extracting', f.name)
with gzip.GzipFile(fileobj=f) as bytestream:
magic = _read32(bytestream)
if magic != 2051:
raise ValueError('Invalid magic number %d in MNIST image file: %s' %
(magic, f.name))
num_images = _read32(bytestream)
rows = _read32(bytestream)
cols = _read32(bytestream)
buf = bytestream.read(rows * cols * num_images)
data = numpy.frombuffer(buf, dtype=numpy.uint8)
data = data.reshape(num_images, rows, cols, 1)
return data
@deprecated(None, 'Please use tf.one_hot on tensors.')
def _dense_to_one_hot(labels_dense, num_classes):
"""Convert class labels from scalars to one-hot vectors."""
num_labels = labels_dense.shape[0]
index_offset = numpy.arange(num_labels) * num_classes
labels_one_hot = numpy.zeros((num_labels, num_classes))
labels_one_hot.flat[index_offset + labels_dense.ravel()] = 1
return labels_one_hot
@deprecated(None, 'Please use tf.data to implement this functionality.')
def _extract_labels(f, one_hot=False, num_classes=10):
"""Extract the labels into a 1D uint8 numpy array [index].
Args:
f: A file object that can be passed into a gzip reader.
one_hot: Does one hot encoding for the result.
num_classes: Number of classes for the one hot encoding.
Returns:
labels: a 1D uint8 numpy array.
Raises:
ValueError: If the bystream doesn't start with 2049.
"""
print('Extracting', f.name)
with gzip.GzipFile(fileobj=f) as bytestream:
magic = _read32(bytestream)
if magic != 2049:
raise ValueError('Invalid magic number %d in MNIST label file: %s' %
(magic, f.name))
num_items = _read32(bytestream)
buf = bytestream.read(num_items)
labels = numpy.frombuffer(buf, dtype=numpy.uint8)
if one_hot:
return _dense_to_one_hot(labels, num_classes)
return labels
class _DataSet(object):
"""Container class for a _DataSet (deprecated).
THIS CLASS IS DEPRECATED.
"""
@deprecated(None, 'Please use alternatives such as official/mnist/_DataSet.py'
' from tensorflow/models.')
def __init__(self,
images,
labels,
fake_data=False,
one_hot=False,
dtype=dtypes.float32,
reshape=True,
seed=None):
"""Construct a _DataSet.
one_hot arg is used only if fake_data is true. `dtype` can be either
`uint8` to leave the input as `[0, 255]`, or `float32` to rescale into
`[0, 1]`. Seed arg provides for convenient deterministic testing.
Args:
images: The images
labels: The labels
fake_data: Ignore inages and labels, use fake data.
one_hot: Bool, return the labels as one hot vectors (if True) or ints (if
False).
dtype: Output image dtype. One of [uint8, float32]. `uint8` output has
range [0,255]. float32 output has range [0,1].
reshape: Bool. If True returned images are returned flattened to vectors.
seed: The random seed to use.
"""
seed1, seed2 = random_seed.get_seed(seed)
# If op level seed is not set, use whatever graph level seed is returned
numpy.random.seed(seed1 if seed is None else seed2)
dtype = dtypes.as_dtype(dtype).base_dtype
if dtype not in (dtypes.uint8, dtypes.float32):
raise TypeError('Invalid image dtype %r, expected uint8 or float32' %
dtype)
if fake_data:
self._num_examples = 10000
self.one_hot = one_hot
else:
assert images.shape[0] == labels.shape[0], (
'images.shape: %s labels.shape: %s' % (images.shape, labels.shape))
self._num_examples = images.shape[0]
# Convert shape from [num examples, rows, columns, depth]
# to [num examples, rows*columns] (assuming depth == 1)
if reshape:
assert images.shape[3] == 1
images = images.reshape(images.shape[0],
images.shape[1] * images.shape[2])
if dtype == dtypes.float32:
# Convert from [0, 255] -> [0.0, 1.0].
images = images.astype(numpy.float32)
images = numpy.multiply(images, 1.0 / 255.0)
self._images = images
self._labels = labels
self._epochs_completed = 0
self._index_in_epoch = 0
@property
def images(self):
return self._images
@property
def labels(self):
return self._labels
@property
def num_examples(self):
return self._num_examples
@property
def epochs_completed(self):
return self._epochs_completed
def next_batch(self, batch_size, fake_data=False, shuffle=True):
"""Return the next `batch_size` examples from this data set."""
if fake_data:
fake_image = [1] * 784
if self.one_hot:
fake_label = [1] + [0] * 9
else:
fake_label = 0
return [fake_image for _ in xrange(batch_size)
], [fake_label for _ in xrange(batch_size)]
start = self._index_in_epoch
# Shuffle for the first epoch
if self._epochs_completed == 0 and start == 0 and shuffle:
perm0 = numpy.arange(self._num_examples)
numpy.random.shuffle(perm0)
self._images = self.images[perm0]
self._labels = self.labels[perm0]
# Go to the next epoch
if start + batch_size > self._num_examples:
# Finished epoch
self._epochs_completed += 1
# Get the rest examples in this epoch
rest_num_examples = self._num_examples - start
images_rest_part = self._images[start:self._num_examples]
labels_rest_part = self._labels[start:self._num_examples]
# Shuffle the data
if shuffle:
perm = numpy.arange(self._num_examples)
numpy.random.shuffle(perm)
self._images = self.images[perm]
self._labels = self.labels[perm]
# Start next epoch
start = 0
self._index_in_epoch = batch_size - rest_num_examples
end = self._index_in_epoch
images_new_part = self._images[start:end]
labels_new_part = self._labels[start:end]
return numpy.concatenate((images_rest_part, images_new_part),
axis=0), numpy.concatenate(
(labels_rest_part, labels_new_part), axis=0)
else:
self._index_in_epoch += batch_size
end = self._index_in_epoch
return self._images[start:end], self._labels[start:end]
@deprecated(None, 'Please write your own downloading logic.')
def _maybe_download(filename, work_directory, source_url):
"""Download the data from source url, unless it's already here.
Args:
filename: string, name of the file in the directory.
work_directory: string, path to working directory.
source_url: url to download from if file doesn't exist.
Returns:
Path to resulting file.
"""
if not gfile.Exists(work_directory):
gfile.MakeDirs(work_directory)
filepath = os.path.join(work_directory, filename)
if not gfile.Exists(filepath):
urllib.request.urlretrieve(source_url, filepath)
with gfile.GFile(filepath) as f:
size = f.size()
print('Successfully downloaded', filename, size, 'bytes.')
return filepath
@deprecated(None, 'Please use alternatives such as:'
' tensorflow_datasets.load(\'mnist\')')
def read_data_sets(train_dir,
fake_data=False,
one_hot=False,
dtype=dtypes.float32,
reshape=True,
validation_size=5000,
seed=None,
source_url=DEFAULT_SOURCE_URL):
if fake_data:
def fake():
return _DataSet([], [],
fake_data=True,
one_hot=one_hot,
dtype=dtype,
seed=seed)
train = fake()
validation = fake()
test = fake()
return _Datasets(train=train, validation=validation, test=test)
if not source_url: # empty string check
source_url = DEFAULT_SOURCE_URL
train_images_file = 'train-images-idx3-ubyte.gz'
train_labels_file = 'train-labels-idx1-ubyte.gz'
test_images_file = 't10k-images-idx3-ubyte.gz'
test_labels_file = 't10k-labels-idx1-ubyte.gz'
local_file = _maybe_download(train_images_file, train_dir,
source_url + train_images_file)
with gfile.Open(local_file, 'rb') as f:
train_images = _extract_images(f)
local_file = _maybe_download(train_labels_file, train_dir,
source_url + train_labels_file)
with gfile.Open(local_file, 'rb') as f:
train_labels = _extract_labels(f, one_hot=one_hot)
local_file = _maybe_download(test_images_file, train_dir,
source_url + test_images_file)
with gfile.Open(local_file, 'rb') as f:
test_images = _extract_images(f)
local_file = _maybe_download(test_labels_file, train_dir,
source_url + test_labels_file)
with gfile.Open(local_file, 'rb') as f:
test_labels = _extract_labels(f, one_hot=one_hot)
if not 0 <= validation_size <= len(train_images):
raise ValueError(
'Validation size should be between 0 and {}. Received: {}.'.format(
len(train_images), validation_size))
validation_images = train_images[:validation_size]
validation_labels = train_labels[:validation_size]
train_images = train_images[validation_size:]
train_labels = train_labels[validation_size:]
options = dict(dtype=dtype, reshape=reshape, seed=seed)
train = _DataSet(train_images, train_labels, **options)
validation = _DataSet(validation_images, validation_labels, **options)
test = _DataSet(test_images, test_labels, **options)
return _Datasets(train=train, validation=validation, test=test)
二、解决报错ModuleNotFoundError: No module named ‘tensorflow.contrib‘
问题:在TensorFlow2.x版本已经不能使用contrib包

三、安装onnx报错assert CMAKE, ‘Could not find “cmake“ executable!‘
经过百度,查得:安装onnx需要protobuf编译所以安装前需要安装protobuf。
四、ImportError: cannot import name 'builder' from 'google.protobuf.internal'
问题:当运行torch转onnx的代码时,出现ImportError: cannot import name 'builder' from 'google.protobuf.internal'
,如下图:
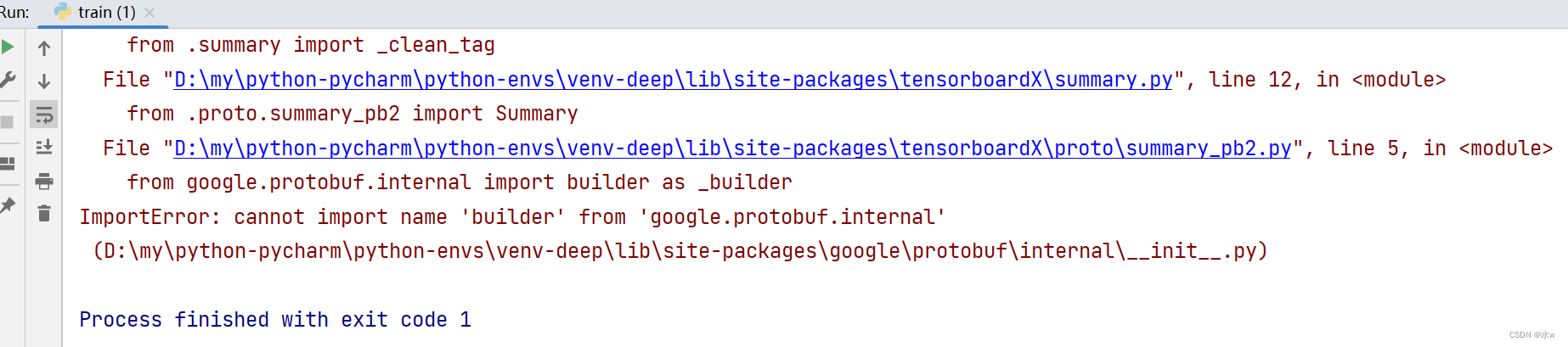
原因:由于使用的google.protobuf
版本太低而引起的。在较新的版本中,builder
模块已经移动到了google.protobuf
包中,而不再在google.protobuf.internal
中。
解决办法:升级protobuf库
pip install --upgrade protobuf
五、解决ModuleNotFoundError: No module named 'sklearn'
问题:sklearn第三方库安装失败
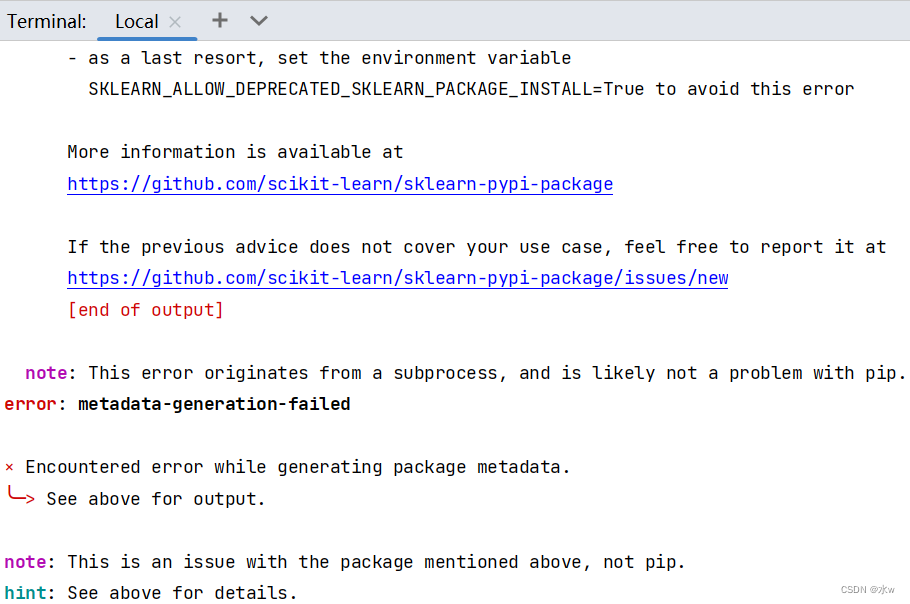
原因:查看别人库的列表,发现sklearn的包名是scikit-learn
解决:安装scikit-learn,
pip install -i https://pypi.tuna.tsinghua.edu.cn/simple scikit-learn
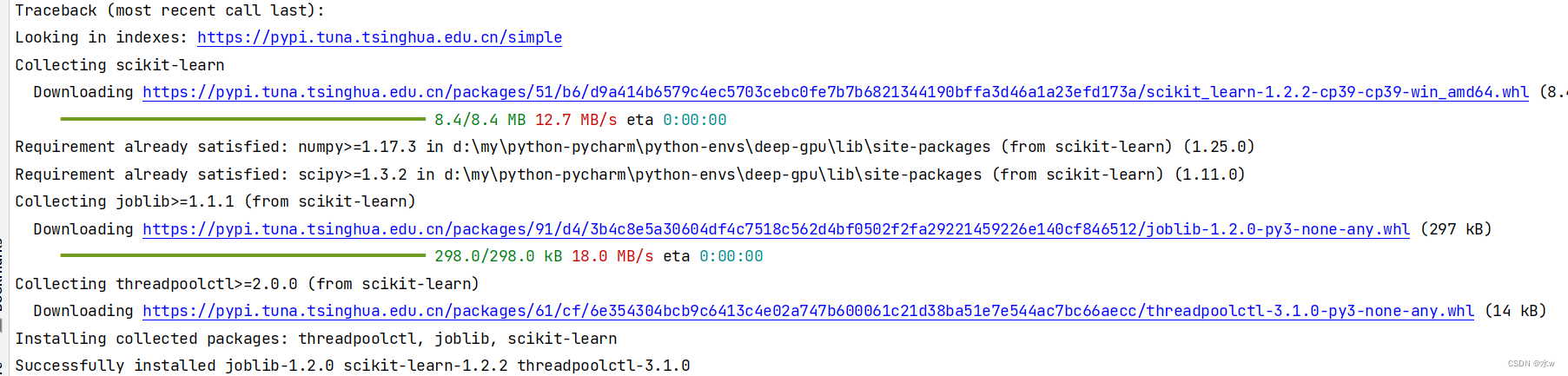
六、解决AttributeError: module ‘torch._C‘ has no attribute ‘_cuda_setDevice‘
网上查询原因:说我安装的torch是适合CPU的,而不是适合GPU的。于是我查询pytorch版本情况,代码如下,
import torch
torch.cuda.is_available()
结果是False。
显而易见,环境使用的是CPU版本的torch,但是我仔细检查了一下我安装的命令,如下
解决:下载三个安装包,适合GPU版本的,
可以参考这篇(1条消息) GPU版本安装Pytorch教程最新方法_pytorch gpu_水w的博客-CSDN博客

然后分别pip install 他们,这样就能够安装适合GPU版本的torch了。
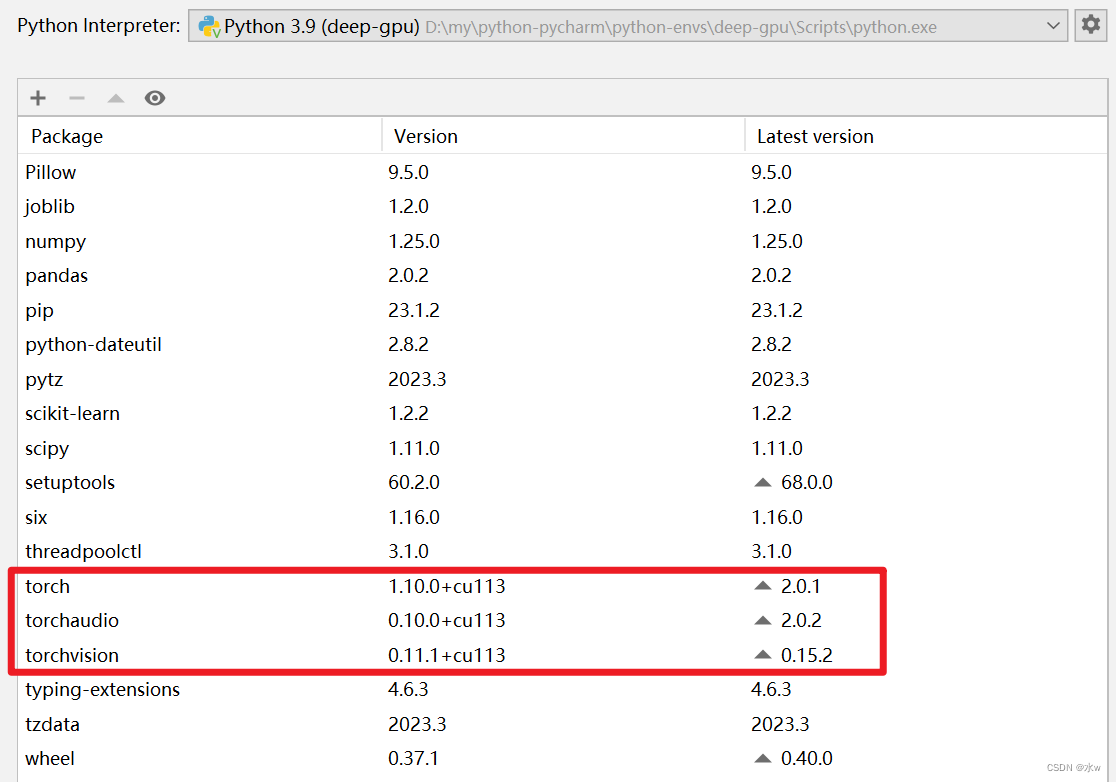
七、解决ImportError: Missing optional dependency 'pytables'. Use pip or conda to install pytables.
问题:运行py文件报错
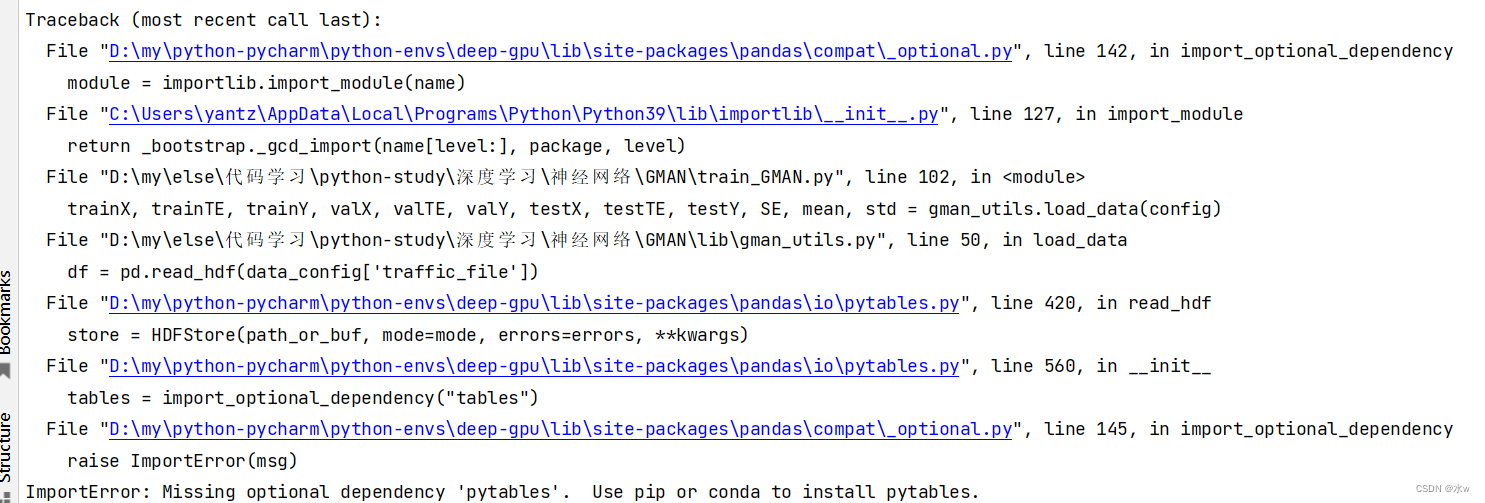
解决历程:按照提示安装pytables,"pip install pytables"安装失败,然后试了"pip install tables"安装上了。
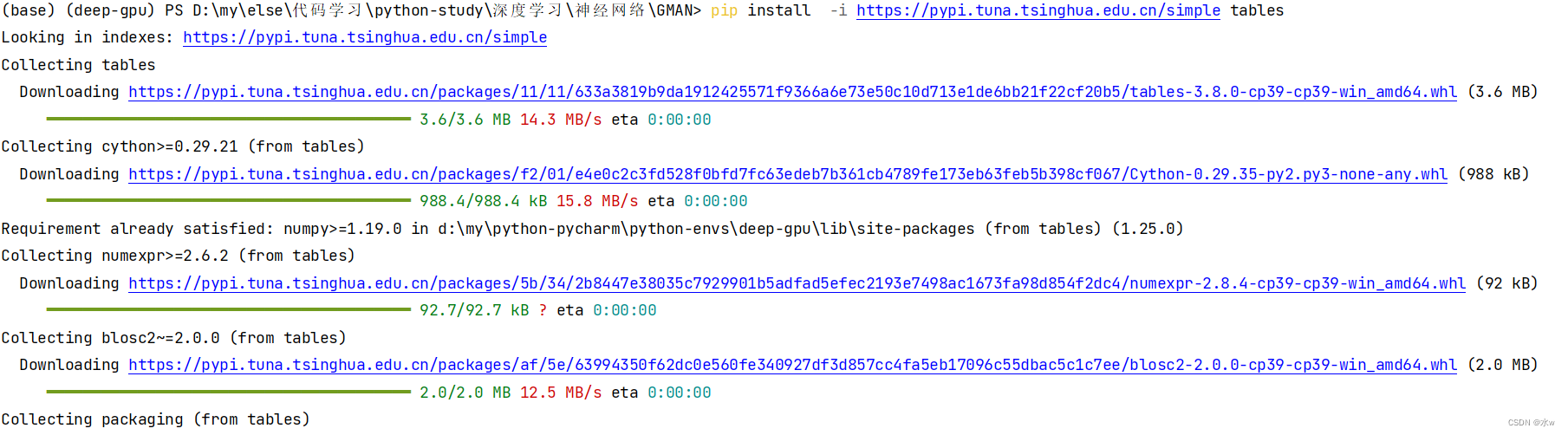
重新运行代码,发现就不报错了。
八、解决AttributeError: module ‘distutils’ has no attribute ‘version’.
问题: AttributeError: module ‘distutils’ has no attribute ‘version’.
解决: setuptools版本问题”,版本过高导致的问题;setuptools版本
- 第一步: pip uninstall setuptools【使用pip,不能使用 conda uninstall setuptools ; 【不能使用conda的命令,原因是,conda在卸载的时候,会自动分析与其相关的库,然后全部删除,如果y的话,整个环境都需要重新配置。
- 第二步: pip或者conda install setuptools==59.5.0【现在最新的版本已经到了65了,之前的老版本只是部分保留,找不到的版本不行
然后重新运行了代码,发现没有报错了。
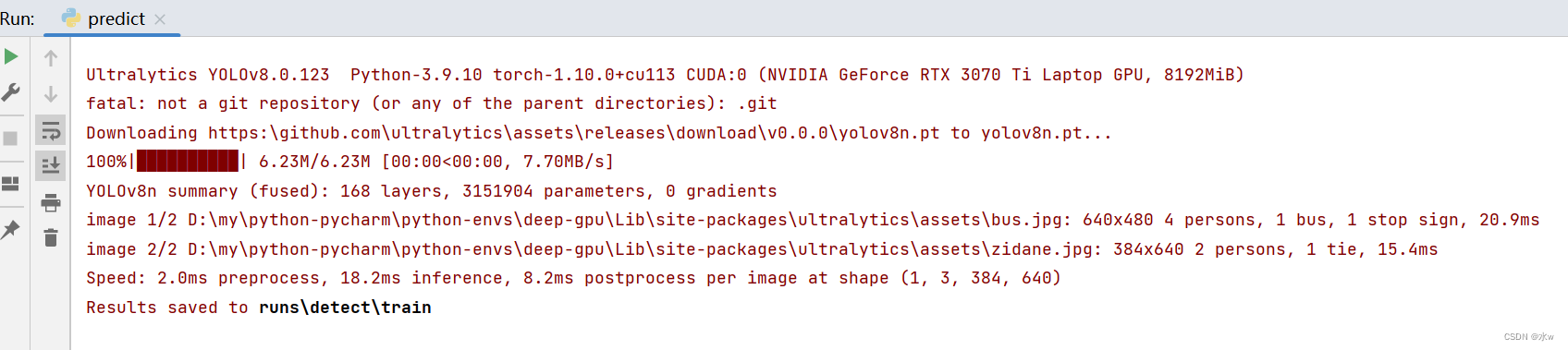