特征工程,使用线性回归机制来拟合非常复杂甚至非线性(存在
x
n
x^n
xn)的函数。
1. 导入
import numpy as np
import matplotlib.pyplot as plt
from lab_utils_multi import zscore_normalize_features, run_gradient_descent_feng
np.set_printoptions(precision=2) # reduced display precision on numpy arrays
2.多项式特征
这是线性回归时使用的
f
w
,
b
=
w
0
x
0
+
w
1
x
1
+
.
.
.
+
w
n
−
1
x
n
−
1
+
b
(1)
f_{\mathbf{w},b} = w_0x_0 + w_1x_1+ ... + w_{n-1}x_{n-1} + b \tag{1}
fw,b=w0x0+w1x1+...+wn−1xn−1+b(1)
先看看按照以前的线性回归方法的效果
# create target data
x = np.arange(0, 20, 1)
y = 1 + x**2
X = x.reshape(-1, 1)
model_w,model_b = run_gradient_descent_feng(X,y,iterations=1000, alpha = 1e-2)
plt.scatter(x, y, marker='x', c='r', label="Actual Value"); plt.title("no feature engineering")
plt.plot(x,X@model_w + model_b, label="Predicted Value"); plt.xlabel("X"); plt.ylabel("y"); plt.legend(); plt.show()
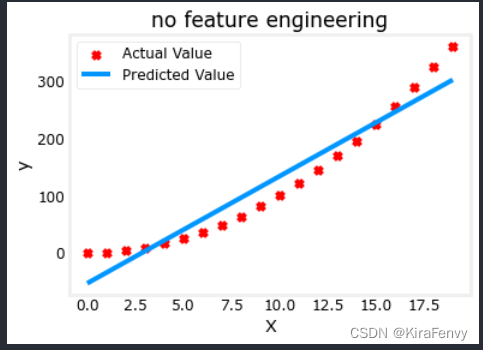
明显不行,我们需要多项式特征,因此我们进行特征工程,调整x的次数
# create target data
x = np.arange(0, 20, 1)
y = 1 + x**2
# Engineer features
X = x**2 #<-- added engineered feature
X = X.reshape(-1, 1) #X should be a 2-D Matrix
model_w,model_b = run_gradient_descent_feng(X, y, iterations=10000, alpha = 1e-5)
Iteration 0, Cost: 7.32922e+03
Iteration 1000, Cost: 2.24844e-01
Iteration 2000, Cost: 2.22795e-01
Iteration 0, Cost: 7.32922e+03
Iteration 1000, Cost: 2.24844e-01
Iteration 2000, Cost: 2.22795e-01
Iteration 3000, Cost: 2.20764e-01
Iteration 4000, Cost: 2.18752e-01
Iteration 5000, Cost: 2.16758e-01
Iteration 3000, Cost: 2.20764e-01
Iteration 4000, Cost: 2.18752e-01
Iteration 5000, Cost: 2.16758e-01
Iteration 6000, Cost: 2.14782e-01
Iteration 7000, Cost: 2.12824e-01
Iteration 8000, Cost: 2.10884e-01
Iteration 6000, Cost: 2.14782e-01
Iteration 7000, Cost: 2.12824e-01
Iteration 8000, Cost: 2.10884e-01
Iteration 9000, Cost: 2.08962e-01
w,b found by gradient descent: w: [1.], b: 0.0490
Iteration 9000, Cost: 2.08962e-01
w,b found by gradient descent: w: [1.], b: 0.0490
plt.scatter(x, y, marker='x', c='r', label="Actual Value"); plt.title("Added x**2 feature")
plt.plot(x, np.dot(X,model_w) + model_b, label="Predicted Value"); plt.xlabel("x"); plt.ylabel("y"); plt.legend(); plt.show()
拟合出来的式子是
y
=
1
∗
x
0
2
+
0.049
y=1*x_0^2+0.049
y=1∗x02+0.049
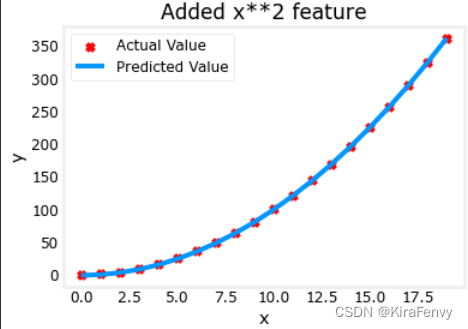
3.特征选择
Above, we knew that an
x
2
x^2
x2 term was required. It may not always be obvious which features are required. One could add a variety of potential features to try and find the most useful. For example, what if we had instead tried :
y
=
w
0
x
0
+
w
1
x
1
2
+
w
2
x
2
3
+
b
y=w_0x_0 + w_1x_1^2 + w_2x_2^3+b
y=w0x0+w1x12+w2x23+b ?
试一下别的,看拟合程度会不会更高
# create target data
x = np.arange(0, 20, 1)
y = x**2
# engineer features .
X = np.c_[x, x**2, x**3] #<-- added engineered feature
model_w,model_b = run_gradient_descent_feng(X, y, iterations=10000, alpha=1e-7)
plt.scatter(x, y, marker='x', c='r', label="Actual Value"); plt.title("x, x**2, x**3 features")
plt.plot(x, X@model_w + model_b, label="Predicted Value"); plt.xlabel("x"); plt.ylabel("y"); plt.legend(); plt.show()
Iteration 0, Cost: 1.14029e+03
Iteration 1000, Cost: 3.28539e+02
Iteration 2000, Cost: 2.80443e+02
Iteration 0, Cost: 1.14029e+03
Iteration 1000, Cost: 3.28539e+02
Iteration 2000, Cost: 2.80443e+02
Iteration 3000, Cost: 2.39389e+02
Iteration 4000, Cost: 2.04344e+02
Iteration 5000, Cost: 1.74430e+02
Iteration 3000, Cost: 2.39389e+02
Iteration 4000, Cost: 2.04344e+02
Iteration 5000, Cost: 1.74430e+02
Iteration 6000, Cost: 1.48896e+02
Iteration 7000, Cost: 1.27100e+02
Iteration 8000, Cost: 1.08495e+02
Iteration 6000, Cost: 1.48896e+02
Iteration 7000, Cost: 1.27100e+02
Iteration 8000, Cost: 1.08495e+02
Iteration 9000, Cost: 9.26132e+01
w,b found by gradient descent: w: [0.08 0.54 0.03], b: 0.0106
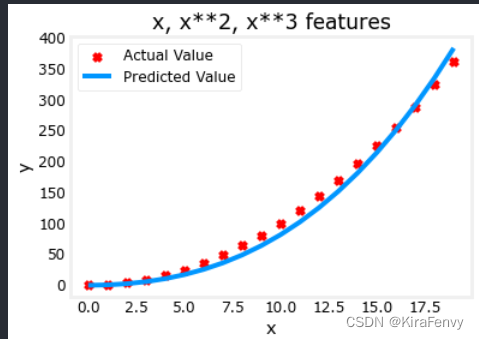
拟合出来的式子:
0.08
x
+
0.54
x
2
+
0.03
x
3
+
0.0106
0.08x + 0.54x^2 + 0.03x^3 + 0.0106
0.08x+0.54x2+0.03x3+0.0106
梯度下降通过强调其相关参数为我们选择“正确”的特征,较小的权重值意味着不太重要/正确的特征
4.多项式特征与线性特征的关联
我们进行多项式回归,也是在选择和y线性关联程度最高的特征
# create target data
x = np.arange(0, 20, 1)
y = x**2
# engineer features .
X = np.c_[x, x**2, x**3] #<-- added engineered feature
X_features = ['x','x^2','x^3']
fig,ax=plt.subplots(1, 3, figsize=(12, 3), sharey=True)
for i in range(len(ax)):
ax[i].scatter(X[:,i],y)
ax[i].set_xlabel(X_features[i])
ax[0].set_ylabel("y")
plt.show()
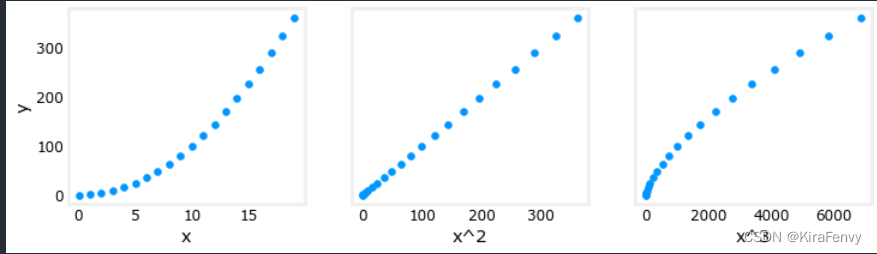
5. 特征缩放 Scaling features
# create target data
x = np.arange(0,20,1)
X = np.c_[x, x**2, x**3]
print(f"Peak to Peak range by column in Raw X:{np.ptp(X,axis=0)}")
# add mean_normalization
X = zscore_normalize_features(X)
print(f"Peak to Peak range by column in Normalized X:{np.ptp(X,axis=0)}")
Peak to Peak range by column in Raw X:[ 19 361 6859]
Peak to Peak range by column in Normalized X:[3.3 3.18 3.28]
Peak to Peak range by column in Raw X:[ 19 361 6859]
Peak to Peak range by column in Normalized X:[3.3 3.18 3.28]
x = np.arange(0,20,1)
y = x**2
X = np.c_[x, x**2, x**3]
X = zscore_normalize_features(X)
model_w, model_b = run_gradient_descent_feng(X, y, iterations=100000, alpha=1e-1)
plt.scatter(x, y, marker='x', c='r', label="Actual Value"); plt.title("Normalized x x**2, x**3 feature")
plt.plot(x,X@model_w + model_b, label="Predicted Value"); plt.xlabel("x"); plt.ylabel("y"); plt.legend(); plt.show()
Iteration 0, Cost: 9.42147e+03
Iteration 0, Cost: 9.42147e+03
Iteration 10000, Cost: 3.90938e-01
Iteration 10000, Cost: 3.90938e-01
Iteration 20000, Cost: 2.78389e-02
Iteration 20000, Cost: 2.78389e-02
Iteration 30000, Cost: 1.98242e-03
Iteration 30000, Cost: 1.98242e-03
Iteration 40000, Cost: 1.41169e-04
Iteration 40000, Cost: 1.41169e-04
Iteration 50000, Cost: 1.00527e-05
Iteration 50000, Cost: 1.00527e-05
Iteration 60000, Cost: 7.15855e-07
Iteration 60000, Cost: 7.15855e-07
Iteration 70000, Cost: 5.09763e-08
Iteration 70000, Cost: 5.09763e-08
Iteration 80000, Cost: 3.63004e-09
Iteration 80000, Cost: 3.63004e-09
Iteration 90000, Cost: 2.58497e-10
Iteration 90000, Cost: 2.58497e-10
w,b found by gradient descent: w: [5.27e-05 1.13e+02 8.43e-05], b: 123.5000
w,b found by gradient descent: w: [5.27e-05 1.13e+02 8.43e-05], b: 123.5000
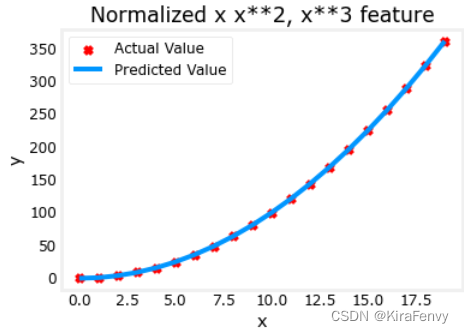
6.复杂函数的拟合
x = np.arange(0,20,1)
y = np.cos(x/2)
X = np.c_[x, x**2, x**3,x**4, x**5, x**6, x**7, x**8, x**9, x**10, x**11, x**12, x**13]
X = zscore_normalize_features(X)
model_w,model_b = run_gradient_descent_feng(X, y, iterations=1000000, alpha = 1e-1)
plt.scatter(x, y, marker='x', c='r', label="Actual Value"); plt.title("Normalized x x**2, x**3 feature")
plt.plot(x,X@model_w + model_b, label="Predicted Value"); plt.xlabel("x"); plt.ylabel("y"); plt.legend(); plt.show()
Iteration 0, Cost: 2.24887e-01
Iteration 0, Cost: 2.24887e-01
Iteration 100000, Cost: 2.31061e-02
Iteration 100000, Cost: 2.31061e-02
Iteration 200000, Cost: 1.83619e-02
Iteration 200000, Cost: 1.83619e-02
Iteration 300000, Cost: 1.47950e-02
Iteration 300000, Cost: 1.47950e-02
Iteration 400000, Cost: 1.21114e-02
Iteration 400000, Cost: 1.21114e-02
Iteration 500000, Cost: 1.00914e-02
Iteration 500000, Cost: 1.00914e-02
Iteration 600000, Cost: 8.57025e-03
Iteration 600000, Cost: 8.57025e-03
Iteration 700000, Cost: 7.42385e-03
Iteration 700000, Cost: 7.42385e-03
Iteration 800000, Cost: 6.55908e-03
Iteration 800000, Cost: 6.55908e-03
Iteration 900000, Cost: 5.90594e-03
Iteration 900000, Cost: 5.90594e-03
w,b found by gradient descent: w: [-1.61e+00 -1.01e+01 3.00e+01 -6.92e-01 -2.37e+01 -1.51e+01 2.09e+01
-2.29e-03 -4.69e-03 5.51e-02 1.07e-01 -2.53e-02 6.49e-02], b: -0.0073
w,b found by gradient descent: w: [-1.61e+00 -1.01e+01 3.00e+01 -6.92e-01 -2.37e+01 -1.51e+01 2.09e+01
-2.29e-03 -4.69e-03 5.51e-02 1.07e-01 -2.53e-02 6.49e-02], b: -0.0073
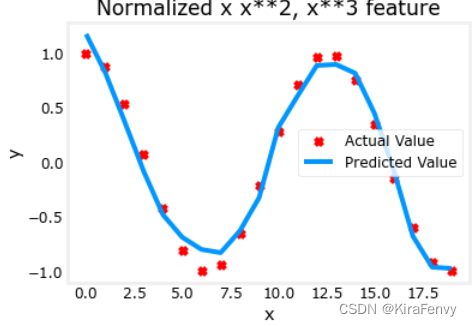
7.课后题
- 特征缩放的时候要减去平均值
- 代价不能较快的减少证明学习率偏大,代价反而增大证明学习率太大
- 当特征之间的具体数值差距过大的时候,需要用到特征缩放
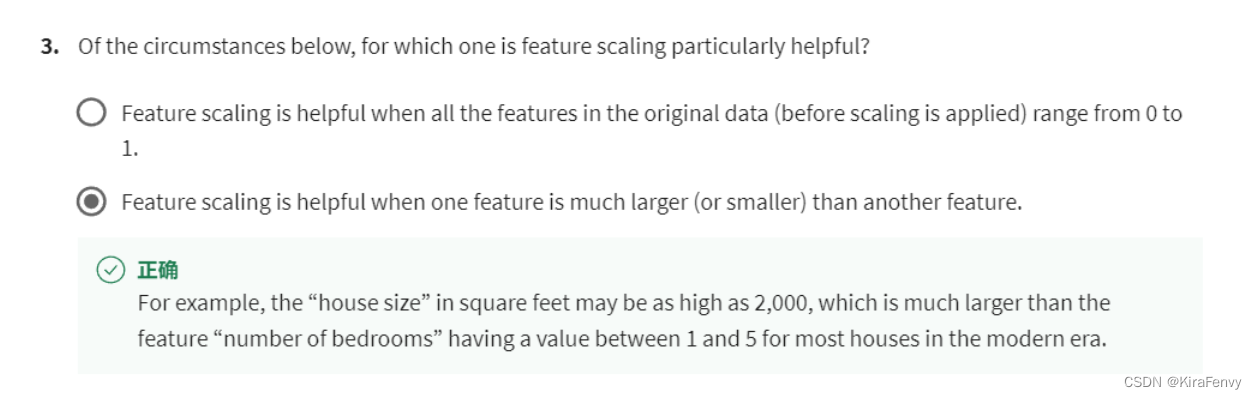
4. 数量乘价格才是销售总价
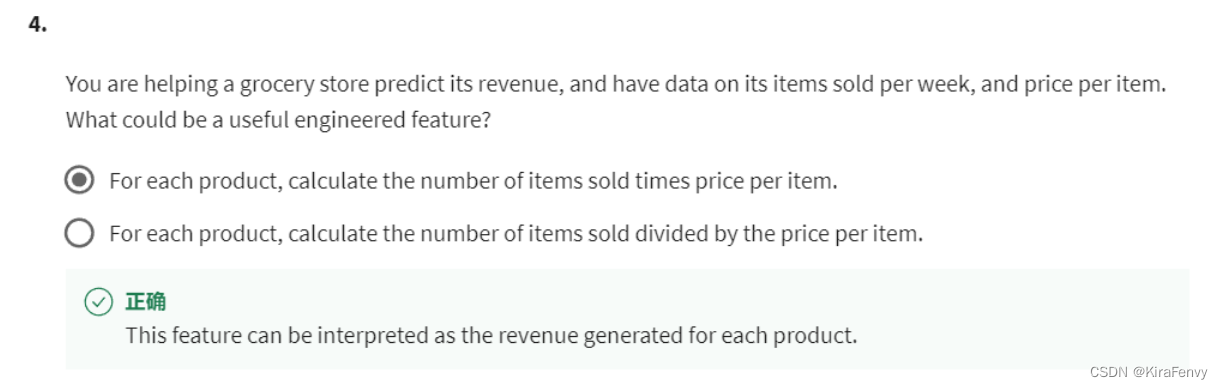